The realm of culinary exploration is vast, and navigating its myriad options can be daunting. Enter the random food place generator, a digital tool designed to alleviate decision fatigue and inject an element of serendipity into your dining experiences. This guide delves into the mechanics, functionalities, and potential of such a generator, exploring its core benefits and target audience.
We will examine how these generators function, from the data they leverage to the algorithms that power their recommendations. Furthermore, the guide explores practical applications, user interface design, and the technical aspects of bringing such a tool to life. We will analyze existing examples and discuss future possibilities, painting a comprehensive picture of this innovative approach to discovering new culinary adventures.
Random Food Place Generator: A Digital Muse for the Hungry
A random food place generator, in its simplest form, is a digital tool designed to select dining establishments at random. It’s a virtual coin toss for the culinary world, offering an element of chance to the often-overwhelming decision of where to eat. This seemingly simple function belies a deeper purpose: to liberate users from the tyranny of choice and to introduce them to new gastronomic experiences they might otherwise miss.
Core Function and Purpose
The primary function of a random food place generator is to provide users with an unbiased selection of restaurants, cafes, or food vendors. Its purpose extends beyond mere randomness; it’s a catalyst for culinary exploration. By eliminating the cognitive load of endless browsing and deliberation, the generator encourages spontaneity and the discovery of hidden gems. It’s a digital compass, pointing towards unknown flavors and unexplored corners of the dining landscape.
Benefits for Users
The benefits for users are manifold, ranging from convenience to the expansion of their palates. These generators offer a sanctuary from the paradox of choice, the psychological distress experienced when faced with too many options. They can also:
- Save Time and Reduce Decision Fatigue: The time spent scrolling through reviews and menus is significantly reduced. The generator provides an instant answer, freeing up valuable time for other pursuits. This is particularly useful for those with busy schedules or who simply prefer to outsource the decision-making process.
- Discover New Culinary Experiences: By venturing beyond familiar choices, users are exposed to a wider range of cuisines, restaurants, and dining experiences. This can lead to the discovery of new favorite foods, cultures, and neighborhoods. Imagine, for example, a user who habitually orders pizza being randomly directed to a hidden Ethiopian restaurant, opening their world to a new spectrum of flavors and textures.
- Enhance Spontaneity and Adventure: The element of surprise inherent in a random selection fosters a sense of adventure and excitement. It encourages users to step outside their comfort zones and embrace the unexpected. This can be especially appealing to those seeking to break free from routine and inject a little unpredictability into their lives.
- Reduce Bias and Promote Fairness: Random generators can help overcome personal biases, such as a preference for a specific cuisine or restaurant type. This can lead to a more balanced and diverse dining experience. For example, a user might consistently choose Italian restaurants due to familiarity. A random generator could push them to explore Vietnamese cuisine, thereby broadening their culinary horizons.
“The best way to predict the future is to create it.” – Peter Drucker (loosely adapted to the context of food)
Target Audience & Use Cases
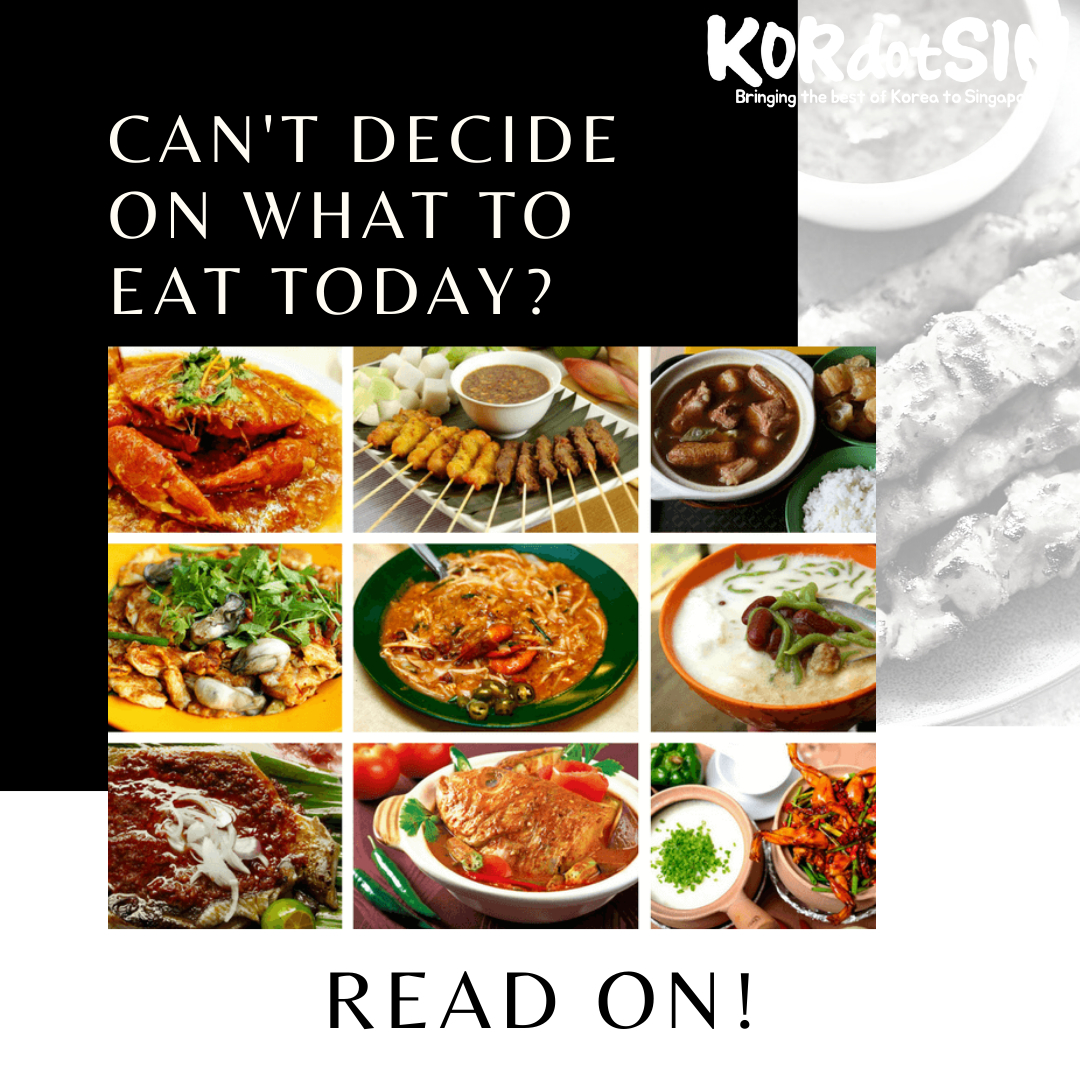
A digital muse, whispering culinary possibilities, the random food place generator finds its solace in the diverse cravings of a hungry world. It’s a tool born from the quiet desperation of decision fatigue, a gentle nudge towards uncharted flavors, a companion in the search for the next delicious memory. Its usefulness transcends the simple act of choosing a meal; it weaves itself into the fabric of our social interactions, our routines, and our moments of solitary indulgence.This generator is more than just a list; it’s an invitation.
For descriptions on additional topics like chinese food pottstown, please visit the available chinese food pottstown.
It opens doors to experiences, encourages exploration, and injects a touch of serendipity into the mundane act of eating. It speaks to the romantic, the pragmatic, and the adventurous within us all.
User Groups
The random food place generator serves a symphony of users, each with their own unique needs and desires. From the solitary diner seeking a novel experience to the family wrestling with conflicting preferences, the generator provides a solution. The following user groups are the most prominent:
- The Solo Adventurer: Embracing the freedom of dining alone, this user seeks variety and a break from routine. They might be new to a city or simply tired of the usual haunts.
- The Couple in Search of Romance: Date night requires a spark. The generator can suggest a place that sets the mood, offering a new experience to share.
- The Family Navigating Diverse Tastes: Pleasing everyone can be a challenge. The generator offers a starting point, suggesting options that cater to different preferences.
- The Busy Professional Seeking Efficiency: Lunch breaks are precious. The generator provides quick options, saving time and mental energy.
- The Group of Friends Yearning for Adventure: Deciding on a restaurant can take forever. The generator cuts through the indecision, prompting spontaneous gatherings.
Use Case Scenarios
The scenarios where the random food place generator shines are as varied as the restaurants it suggests. Consider these examples:
- Date Night Dilemma: “Where should we go tonight?” is a question that can paralyze a date before it even begins. The generator offers a selection of possibilities, injecting excitement and removing the pressure of the “perfect” choice.
- Lunch Break Liberation: The mid-day meal can become a repetitive ritual. The generator provides a quick escape from the office, suggesting a new place to recharge.
- Family Dinner Frustration: Catering to everyone’s tastes can feel impossible. The generator offers a compromise, presenting options that might satisfy the picky eater and the adventurous gourmand alike.
- Weekend Exploration: When the week is over and the city is calling, the generator offers a sense of discovery, prompting you to venture to a neighborhood or type of cuisine you may not have considered.
Use Case Table
The following table presents a comprehensive overview of the various use cases and their corresponding user types, emphasizing how the generator caters to their unique needs.
User Type | Use Case | Generator Benefit | Example Scenario |
---|---|---|---|
Solo Adventurer | Discovering New Restaurants | Expands culinary horizons and avoids routine. | A solo traveler in a new city uses the generator to find a hidden gem for dinner. |
Couple | Planning a Date Night | Adds spontaneity and removes decision fatigue. | A couple struggling to decide where to go on their anniversary uses the generator to find a romantic restaurant. |
Family | Choosing a Family Dinner Location | Offers a compromise and caters to diverse tastes. | A family with children uses the generator to find a restaurant that offers something for everyone. |
Busy Professional | Quick Lunch Break Selection | Saves time and mental energy during a hectic workday. | A professional uses the generator to quickly find a lunch spot near the office. |
Group of Friends | Spontaneous Gatherings | Eliminates indecision and encourages exploration. | A group of friends uses the generator to decide where to meet for dinner on a Friday night. |
Functionality and Features
The digital hunger, a constant ache in the modern soul, demands a swift and decisive remedy. A random food place generator, in its silent code, offers solace, a whisper of culinary possibility in the vast digital desert. Its features, carefully crafted, are the compass guiding the lost and hungry to their next fleeting pleasure.
Essential Features
The heart of the generator lies in its essential features, the very essence of its being. These are the building blocks of serendipitous feasting.
- Database of Food Places: A meticulously curated collection of restaurants, cafes, and eateries, the lifeblood of the generator. This database should encompass a wide variety of cuisines, price ranges, and locations, constantly updated to reflect the ever-changing culinary landscape. It should include, but not be limited to, the restaurant’s name, address, phone number, website (if available), operating hours, a brief description, and cuisine type.
This database is the soul of the generator.
- Location Services: The ability to pinpoint the user’s current location or accept a user-defined location. This is essential for providing relevant recommendations, ensuring the suggested food places are within a reasonable distance. This feature is the anchor, tethering the digital to the real.
- Filtering Options: The capacity to filter results based on various criteria, such as cuisine type (e.g., Italian, Mexican, Thai), price range (e.g., $, $$, $$$), dietary restrictions (e.g., vegetarian, vegan, gluten-free), and rating (e.g., based on user reviews or other sources). This offers personalization, a tailored experience for each weary traveler.
- Randomization Algorithm: The core engine, the heart of the matter. This algorithm selects food places randomly, based on the user’s preferences and the available data. It should incorporate a degree of weighting to avoid truly obscure choices, while still allowing for genuine surprise. This is the element of chance, the unpredictable whisper of fate.
- User Interface (UI): A clean, intuitive, and user-friendly interface. The UI should be easy to navigate, allowing users to quickly input their preferences and view the recommendations. It should present the information in a clear and concise manner, with visually appealing elements. This is the face of the generator, the first impression.
- Integration with Mapping Services: Seamless integration with mapping services (e.g., Google Maps, Apple Maps). This allows users to easily view the recommended food place on a map and get directions. This is the bridge, connecting the recommendation to reality.
- Review and Rating System: A mechanism for users to review and rate the recommended food places. This provides valuable feedback, allowing the generator to learn and improve over time. It is the echo of experience, shaping the future.
Recommendation Determination
The process of determining a food place recommendation is a dance of data and chance, a delicate balance of logic and whimsy. It’s a whisper in the algorithm’s ear.The generator’s decision-making process is guided by a combination of factors, each playing a crucial role in shaping the final recommendation.
- User Input: The process begins with the user’s input. This includes the user’s location, any filtering criteria they have selected (e.g., cuisine type, price range), and any pre-existing preferences stored in their profile.
- Database Query: The generator queries its database, filtering the food places based on the user’s input. This narrows down the possibilities, creating a subset of eligible restaurants.
- Randomization: Within the filtered subset, the randomization algorithm is applied. This algorithm selects one or more food places randomly. The algorithm may incorporate weighting based on factors such as popularity (e.g., based on reviews), proximity to the user, or the freshness of the data.
- Recommendation Presentation: The selected food place(s) are then presented to the user, along with relevant information such as the name, address, phone number, website (if available), a brief description, and a map.
- Feedback Loop: The generator learns from user feedback, such as reviews and ratings. This feedback is used to refine the algorithm and improve the quality of future recommendations.
The core of the recommendation process can be represented by the following simplified formula:
Recommendation = f(User Input, Database, Randomization Algorithm, Feedback)
Where ‘f’ represents the function that combines these factors.
Process Flowchart
A visual representation of the recommendation generation process reveals the steps involved, a map of the algorithm’s journey.
+---------------------+ +---------------------+ +---------------------+ +---------------------+ +---------------------+ | Start: User Input |------>| Database Query: |------>| Randomization: |------>| Recommendation |------>| End: User Feedback | | (Location, Filters) | | Filter Food Places | | Select Food Place(s) | | Presentation | | (Reviews, Ratings) | +---------------------+ +---------------------+ +---------------------+ +---------------------+ +---------------------+ ^ | | | +---------------------------------+ | | +-----------------------------------------------------------------------+ | | | Feedback Loop: Algorithm Refinement based on User Feedback | | | +-----------------------------------------------------------------------+
This flowchart illustrates the sequential steps, from user input to the final presentation of the recommendation and the crucial feedback loop.
The loop is a constant cycle of learning and adaptation. The database query narrows the field, randomization selects the final choice, and user feedback refines the process, making each recommendation a little more perfect, a little closer to the user’s hidden desires.
Data Sources & Algorithms
A digital whisper, a hungry heart’s solace, the Random Food Place Generator draws sustenance from a world overflowing with culinary whispers. It navigates the labyrinth of data, seeking the perfect confluence of flavors, proximity, and desire. The algorithms, like ancient cartographers, chart the course from craving to consumption.
Types of Data
The generator’s spectral eye gathers its knowledge from disparate realms, weaving a tapestry of information to conjure the perfect meal. It is a collector of fleeting tastes and permanent impressions.
- Restaurant Reviews: The collective voice of the diners, a chorus of praise and lament. Platforms like Yelp, Google Reviews, and TripAdvisor provide star ratings, written reviews, and sentiment analysis. These sources reveal not just the quality of food, but also the atmosphere, service, and overall experience. The average rating acts as a primary indicator of restaurant popularity and quality, informing the initial selection process.
- Location Data: The ever-present anchor, the GPS coordinates that bind us to the physical world. Utilizing services like Google Maps and OpenStreetMap, the generator determines the user’s location and identifies restaurants within a specified radius. This data is crucial, as a restaurant’s appeal diminishes quickly with distance. Consider a scenario: a user is located in downtown Chicago, the generator will prioritize restaurants within a 5-mile radius.
- Cuisine Types: The very essence of culinary diversity, a spectrum of flavors from around the globe. Data on cuisine types is often derived from restaurant categorization on review platforms, restaurant websites, and menu analysis. This enables the generator to match the user’s stated preferences or, in the case of random selection, to introduce unexpected culinary adventures. For example, a user might be craving Italian food, which the generator then uses to filter for restaurants with pasta, pizza, and other Italian dishes.
- Menu Data: The window to a restaurant’s soul, the list of dishes that define its identity. Menu data, gathered from restaurant websites, online ordering platforms (like Grubhub or Uber Eats), and even third-party menu aggregators, offers insights into specific dishes, ingredients, and pricing. This is used to refine recommendations based on dietary restrictions and specific cravings. If a user is vegetarian, the generator will filter for restaurants offering vegetarian options, based on menu data.
- Restaurant Attributes: The subtle details that color the dining experience. Information about restaurant attributes includes price range ($, $$, $$$), ambiance (e.g., romantic, casual, family-friendly), operating hours, and whether the restaurant offers delivery or takeout. These attributes further refine recommendations to meet specific user needs. For example, a user looking for a late-night snack can use the generator to filter for restaurants that are open late and offer delivery.
Algorithms for Restaurant Selection and Ranking
The heart of the generator beats with algorithmic precision, orchestrating the selection and ranking of restaurants. These algorithms are the unseen hands that guide the user towards gastronomic bliss.
The core algorithm often employs a combination of techniques:
- Collaborative Filtering: This approach analyzes the preferences of other users with similar tastes. If a user has previously enjoyed Italian restaurants with high ratings, the algorithm will recommend similar establishments.
- Content-Based Filtering: This method analyzes the features of restaurants themselves, such as cuisine type, price range, and location, to make recommendations.
- Hybrid Filtering: This combines the strengths of both collaborative and content-based filtering, providing more accurate and personalized recommendations.
- Ranking: A ranking algorithm, often a variation of a scoring function, is used to order the restaurants based on a combination of factors. This could involve weighting factors such as:
- Review Score: The average rating of the restaurant, derived from user reviews.
- Relevance: How well the restaurant’s cuisine matches the user’s stated preferences.
- Proximity: The distance between the user and the restaurant.
- Popularity: Based on the number of reviews or the frequency of visits (anonymized).
- Recency: How recently the restaurant has been reviewed or updated (e.g., menus or hours).
The formula for calculating the final score could be:
Final Score = (Review Score
- Weight_Review) + (Relevance
- Weight_Relevance) + (Proximity
- Weight_Proximity) + (Popularity
- Weight_Popularity) + (Recency
- Weight_Recency)
(Weights can be adjusted based on the user’s preferences and the algorithm’s tuning.)
Filtering Options
The generator offers a palette of filters, allowing the user to paint their perfect dining experience. These filters are the tools of customization, transforming the random into the relevant.
- Price Range: From the humble street vendor to the opulent Michelin-starred establishment, the generator allows the user to specify their budget. Options might include “$ (budget-friendly)”, “$$ (moderate)”, and “$$$ (expensive)”.
- Distance: The radius of exploration, the boundaries of the user’s culinary quest. Users can set a maximum distance from their current location, preventing recommendations that are too far to travel. Options might include “Within 1 mile”, “Within 5 miles”, and “Any distance”.
- Cuisine Type: The gateway to a specific flavor profile. The generator allows users to select their preferred cuisine, whether it’s Italian, Mexican, Thai, or something more exotic.
- Dietary Restrictions: Catering to the needs of all diners. The generator allows users to filter for restaurants that offer vegetarian, vegan, gluten-free, or other dietary options.
- Rating: The collective judgment of the masses. Users can specify a minimum star rating to ensure they are only presented with highly-rated restaurants. For example, “4 stars and above”.
- Ambiance: Setting the mood. Filters can include options for “Romantic”, “Casual”, “Family-friendly”, or “Outdoor seating”.
- Open Now: The immediacy of satisfaction. This filter shows only restaurants that are currently open, catering to the immediate needs of a hungry user.
- Delivery/Takeout: Convenience at its finest. This filter focuses on restaurants that offer delivery or takeout options, perfect for those who prefer to dine at home.
User Interface (UI) and User Experience (UX)
The digital muse, a phantom limb for the hungry soul, finds its form in the dance of pixels. The interface, a window to culinary chance, must be a solace, not a source of digital angst. Its beauty lies not in ostentation, but in the quiet efficiency of its design, a whisper of possibility against the clamor of daily life. Let us craft this window, this portal to unknown flavors, with care and a touch of melancholy grace.
Ideal User Interface for a Random Food Place Generator
The ideal interface is a sanctuary of simplicity, a haven from the cluttered landscapes of the digital world. It should be a space where the user feels understood, guided by an intuitive flow that anticipates their needs, a gentle current leading them towards the unknown.
- Minimalist Design: A clean aesthetic, free from unnecessary distractions, allows the user to focus on the core function: discovery. The color palette should be soothing, evoking a sense of calm and anticipation. Think muted tones, perhaps a palette inspired by twilight or the soft glow of a streetlamp on a rainy night.
- Intuitive Navigation: The user should be able to understand and operate the interface without any instructions. The core functionality, such as the “Generate” button, should be prominently displayed and easily accessible.
- Location Input: A prominent search bar or a map integration should allow users to easily specify their desired location. Autofill suggestions based on user location or popular areas would be beneficial.
- Filtering Options: Users should be able to refine their search based on dietary restrictions (vegetarian, vegan, gluten-free), cuisine type, price range, and desired atmosphere (casual, romantic, family-friendly). These options should be presented in a clear and concise manner, perhaps using a series of toggles or dropdown menus.
- Results Display: The generated results should be presented in an easily digestible format, with each entry including the restaurant’s name, address, brief description, and a visual element (e.g., a small image or map pin).
- Integration with External Services: The interface should seamlessly integrate with mapping services (Google Maps, Apple Maps) for directions, and potentially with review platforms (Yelp, Google Reviews) to provide users with additional information.
Best Practices for a Positive User Experience
A positive user experience is the echo of a well-crafted design, a silent promise of satisfaction. It’s about anticipating the user’s needs, removing friction, and creating a sense of delight in the process of discovery.
- Fast Loading Times: Slow loading times can be a source of frustration. Optimize the interface for speed to ensure a seamless experience. Utilize caching techniques and minimize the size of images and other assets.
- Mobile Responsiveness: The interface should be fully responsive and adaptable to different screen sizes, allowing users to access the generator on their smartphones, tablets, and computers.
- Clear and Concise Language: Use simple, straightforward language throughout the interface. Avoid jargon and technical terms.
- Error Handling: Provide clear and helpful error messages if the user encounters any issues. For example, if no restaurants are found within the specified criteria, offer alternative suggestions or allow the user to broaden their search.
- Personalization: Consider incorporating personalization features, such as saving user preferences or allowing users to rate and review the generated restaurants. This would enhance engagement and create a more tailored experience.
- Accessibility: Ensure the interface is accessible to users with disabilities. This includes providing alternative text for images, using sufficient color contrast, and ensuring keyboard navigation.
Mock-up for the Generator’s Interface
Imagine a canvas, a digital twilight. In the upper left corner, a delicate logo: a stylized fork and knife intertwined, bathed in a soft, ethereal glow. The background is a gradient, transitioning from a deep indigo at the top to a pale lavender at the bottom, like the fading light of a summer evening.
The main elements are laid out as follows:
|
Location Input: A prominent search bar with a placeholder text “Enter your location or allow location access” sits just below the logo. An icon representing a location pin is to the right of the search bar. Filtering Options: Below the search bar, a series of toggles or dropdown menus. Examples are “Cuisine Type” (with options like Italian, Mexican, Asian), “Dietary Restrictions” (vegetarian, vegan, gluten-free), and “Price Range” ($, $$, $$$). Each option is accompanied by a subtle icon. Generate Button: A large, circular button in the center of the screen, with the word “Generate” in a soft, inviting font. The button is slightly elevated, creating a sense of depth. Results Display: Below the generate button, an area to display the results. Each result is presented as a card, with the restaurant’s name, a small image, a short description, and a button to view on a map (using an icon of a map pin). The cards have rounded corners and a subtle shadow. |
This mock-up emphasizes simplicity and visual appeal, creating an interface that is both functional and aesthetically pleasing. The design aims to minimize distractions, guiding the user towards the core function of the generator: the delightful discovery of a new culinary experience. The interface evokes a sense of quiet anticipation, a gentle invitation to explore the unknown. This design will provide a simple and pleasing user experience, encouraging exploration and discovery.
The use of a minimalist design, combined with the careful selection of colors and typography, will create an interface that is both beautiful and functional.
Implementation Methods
The digital echo of hunger, a symphony of code and server whispers, must find its vessel. The random food place generator, a fragile dream, takes form in the stark reality of platforms and technologies. Each choice, a subtle brushstroke on the canvas of user experience, carries the weight of potential and the shadow of limitations. We build, we choose, and in the quiet hum of processing, we hope.
Platforms for Deployment
The choice of platform dictates the accessibility and reach of our digital muse. Each offers a unique landscape of possibilities and constraints, shaping the user’s interaction with the generator. The decision, a delicate dance between ambition and practicality, determines where the hungry soul finds solace.
- Web Applications: A versatile and widely accessible option. They offer cross-platform compatibility, reachable from any device with a web browser. The user experience can be rich and interactive, allowing for complex features and dynamic content updates.
- Mobile Applications (iOS and Android): Native mobile apps provide a dedicated and often more responsive user experience. They can leverage device-specific features like location services, push notifications, and offline access. Development, however, requires separate codebases for each platform, increasing development time and cost.
- Desktop Applications: Less common, but viable for specialized use cases or where offline functionality is paramount. They offer potentially greater performance and access to system resources, but suffer from limited portability and distribution challenges.
Technologies and Programming Languages, Random food place generator
The tools of creation, the languages of the digital heart, shape the very essence of the generator. The choice of technology dictates the efficiency, maintainability, and scalability of the project. It’s the whisper of logic that brings the dream to life.
- Frontend Technologies:
- HTML, CSS, and JavaScript: The fundamental building blocks of web applications. HTML structures the content, CSS styles its appearance, and JavaScript adds interactivity and dynamic behavior. Frameworks like React, Angular, or Vue.js can streamline development and enhance user experience.
- Example: Imagine a website where the food place information appears in a dynamic and responsive format. JavaScript, with its event listeners, would be the invisible hand behind the scenes, updating the content whenever the user requests a new suggestion. The user interface, carefully crafted with CSS, presents the information in an aesthetically pleasing and easily understandable way.
- Backend Technologies:
- Programming Languages: Python, Java, Node.js (JavaScript runtime), and Ruby are popular choices for server-side logic. They handle data processing, API interactions, and database management.
- Frameworks: Frameworks like Django (Python), Spring (Java), Express.js (Node.js), and Ruby on Rails can accelerate development and provide robust features.
- Example: Consider the generator pulling data from multiple APIs and databases. The backend, written in Python with a framework like Django, would orchestrate the data retrieval, cleaning, and filtering. The framework handles the complexities of routing requests, managing user authentication, and interacting with the database, ensuring a smooth and efficient operation.
- Database Technologies:
- Relational Databases (SQL): PostgreSQL, MySQL, and SQLite are suitable for structured data storage, ensuring data integrity and consistency.
- NoSQL Databases: MongoDB and Cassandra offer flexibility and scalability for handling large datasets and evolving data structures.
- Example: If the generator needs to store information about food places, user preferences, and search history, a relational database like PostgreSQL might be ideal. The structured nature of SQL databases ensures data integrity and enables efficient querying.
- API Integration: The generator relies heavily on APIs to access food place data, map services, and other relevant information. RESTful APIs are commonly used for communication between the frontend, backend, and external services.
- Cloud Platforms: Services like Amazon Web Services (AWS), Google Cloud Platform (GCP), and Microsoft Azure provide infrastructure for hosting the application, managing databases, and scaling resources.
Platform Pros and Cons
Each platform offers a unique balance of advantages and disadvantages, impacting the overall project. Understanding these trade-offs is crucial for making informed decisions.
- Web Applications:
- Pros: Cross-platform compatibility (accessible on any device with a web browser), easy deployment and updates, wider reach, and potentially lower development costs compared to native mobile apps.
- Cons: Reliance on internet connectivity, potentially less responsive user experience compared to native apps, and limitations in accessing device-specific features.
- Mobile Applications (iOS and Android):
- Pros: Native performance and responsiveness, access to device-specific features (location, camera, etc.), offline functionality, and push notifications.
- Cons: Higher development costs (separate codebases for each platform), platform-specific code, more complex deployment and update processes, and potential app store approval delays.
- Desktop Applications:
- Pros: Potentially better performance and access to system resources, offline functionality, and suitable for specific niche use cases.
- Cons: Limited portability, distribution challenges, platform-specific code, and declining popularity compared to web and mobile apps.
Customization and Personalization
The digital muse, a cold mirror reflecting the yearning of the hungry soul. It offers a feast of choices, a symphony of flavors, yet without the warmth of a knowing touch, the echo of a shared preference. To truly serve, the generator must learn, must remember, must whisper secrets tailored to the individual palate, the ephemeral desires of the moment.
It must transform from a mere suggestion engine into a companion, a silent guide through the labyrinth of culinary possibilities.The path to true personalization lies not in the cold logic of algorithms alone, but in the gentle art of understanding. It requires a willingness to listen, to adapt, to evolve with the user’s changing tastes, the shifting tides of hunger and craving.
This is where the machine transcends its code and begins to approach the realm of empathy, offering not just food, but an experience.
Personalizing Recommendations
The generator’s recommendations, initially a vast, undifferentiated ocean of options, can be sculpted by the user’s hand. This personalization, the key to a meaningful experience, demands a multifaceted approach, a careful orchestration of data and intuition.
-
Preference Profiles: The cornerstone of personalization, allowing users to define their culinary landscape. These profiles would store details such as:
- Dietary Restrictions: Vegan, vegetarian, gluten-free, allergies, and other critical information. This ensures recommendations align with safety and ethical choices.
- Cuisine Preferences: Italian, Mexican, Thai, or more nuanced categories such as ‘spicy’, ‘comfort food’, or ‘healthy’. This is a crucial aspect of the personalization process.
- Price Range: Budget-conscious or willing to splurge, the generator adjusts its suggestions accordingly.
- Location Radius: Restricting the search to a comfortable distance from the user’s current or preferred location.
- Specific Dishes: A list of favorite dishes, ingredients, or restaurants, acting as a starting point for future exploration.
The profile should be easily editable, allowing for the user’s tastes to evolve over time.
- Implicit Learning: Observing user behavior without direct input. The generator can track:
- Search History: What places the user has searched for, even if they didn’t ultimately choose them. This reveals hidden interests.
- Recommendation Interactions: Which recommendations were clicked, viewed, or dismissed. This provides immediate feedback on what resonates with the user.
- Time of Day: The generator can learn that a user typically craves coffee at 8 AM and pizza at 8 PM.
This passive data collection provides insights into the user’s subconscious desires, adding another layer of precision to the recommendations.
- Explicit Feedback: The most direct form of personalization, allowing users to actively shape the recommendations. This involves:
- “Like” and “Dislike” Buttons: Simple, immediate feedback on individual recommendations.
- Ingredient Exclusion: Users can specify ingredients they dislike or are allergic to, refining the search results.
- Rating System: A star rating system allows users to rate the quality of the restaurant, and the food quality.
This feedback, when combined with implicit learning, provides the most comprehensive view of the user’s preferences.
Saving Preferences and Past Choices
Memory, a fragile yet essential component of human experience, must be mirrored in the digital realm. The generator’s ability to remember and recall the user’s past actions creates a sense of continuity, a personalized narrative of culinary exploration.
- Preference Storage: All preference profiles, dietary restrictions, cuisine choices, and price ranges should be securely stored. This allows users to quickly re-establish their preferred settings upon each use. This information is protected.
- History of Choices: A comprehensive history of all past recommendations and selections, including the date, time, and location of each choice. This acts as a digital scrapbook of culinary adventures.
- Favorites List: A dedicated section where users can save their favorite restaurants or dishes for easy access in the future.
- Saved Searches: Allow users to save specific search parameters (e.g., “Italian near me, under $20”) for quick access.
This ability to save and recall is a crucial step towards establishing a lasting relationship between the user and the generator. The user should feel as though the system knows them.
Rating and Feedback Mechanisms
The user’s voice, a whisper in the vastness of the digital landscape, must be heard and heeded. Feedback mechanisms transform the generator from a passive provider into an active listener, constantly refining its understanding of the user’s needs and desires.
- Rating System: A star rating system (e.g., 1-5 stars) allows users to rate the quality of the restaurant, the food, and the overall experience.
- Review Section: A dedicated space for users to write detailed reviews, sharing their experiences, highlighting specific dishes, or offering constructive criticism.
-
Feedback Forms: Optional feedback forms can be used to collect more specific information, such as:
- Was the recommendation accurate?
- Did the restaurant meet the user’s expectations?
- What could be improved?
- Sentiment Analysis: Implementing natural language processing (NLP) techniques to analyze the sentiment of user reviews. This provides a more nuanced understanding of the user’s experience, going beyond simple ratings.
“The hunger of the soul is not merely for food, but for understanding, for a connection, for a taste of something real.”
Integration with Other Services: Random Food Place Generator
The digital wind whispers of convenience, a phantom limb reaching for seamless experiences. Our Random Food Place Generator, a lonely lighthouse in the vast ocean of culinary choice, yearns for connection, for the embrace of other services that can elevate its solitary function to a symphony of possibilities. The integration with external platforms is not merely an enhancement; it is a transfusion of life, breathing vitality into the very core of its existence, transforming a simple suggestion into a tangible reality, a feast for the senses.The integration of these services is akin to adding stars to the night sky, each point of light illuminating the user’s journey, guiding them through the labyrinth of options with an ethereal grace.
This enhances the user’s experience by bridging the gap between the digital suggestion and the physical experience, making the transition from “what to eat” to “where and how to eat it” a fluid and effortless dance. It saves precious time, reduces cognitive load, and adds a layer of delight to the often-stressful task of choosing a meal.
Mapping Services Integration
The integration with mapping services provides a crucial bridge between the digital and the physical realms. It transforms the abstract suggestion of a food place into a concrete location, making it easier for users to visualize and plan their visit.The benefits are manifold.
- Visual Navigation: Users can instantly see the location of the recommended food place on a map, understanding its proximity to their current location or other points of interest. This eliminates the need to manually search for the address and switch between applications.
- Route Planning: Integration allows for automatic route generation, providing directions via various modes of transport (walking, driving, public transit). This streamlines the planning process, allowing users to see estimated travel times and choose the most convenient route.
- Exploration: The map interface can also be used to explore the surrounding area, discovering other restaurants or points of interest nearby, enriching the user’s dining experience.
- Real-time Information: Some mapping services provide real-time traffic updates, which can be incorporated to help users make informed decisions about when to leave and avoid potential delays.
Reservation System Integration
Integration with reservation systems removes the final barrier to enjoying the suggested meal, offering convenience and ensuring a table at the chosen establishment.The advantages are clear:
- Seamless Booking: Users can book a table directly from the generator’s interface, without having to switch to a separate app or website.
- Real-time Availability: Integration with the reservation system provides real-time information on table availability, allowing users to choose a time that suits their schedule.
- Reduced Wait Times: By making a reservation, users can often avoid long wait times, ensuring a smoother and more enjoyable dining experience.
- Personalized Preferences: Integration may allow for the inclusion of personal preferences such as seating location (e.g., outdoor, by the window) or dietary restrictions, enhancing the overall dining experience.
Ordering and Delivery Services Integration
This integration option caters to the user’s desire for convenience and choice, particularly during times when dining out is not feasible.The key advantages are:
- Ordering Convenience: Users can place an order directly from the generator’s interface, eliminating the need to navigate multiple websites or apps.
- Delivery Options: Integration with delivery services provides options for home or office delivery, broadening the scope of usage.
- Menu Exploration: Access to the restaurant’s menu allows users to explore options, customize their orders, and see prices before committing to a meal.
- Payment Processing: Integrated payment systems streamline the ordering process, allowing for secure and convenient payment.
“The algorithm whispered ‘Taverna’s Delight,’ a name like a forgotten song. But the map bloomed, showing the winding streets, the sun setting just so, and the estimated travel time – a mere 15 minutes. Then, the reservation button pulsed, a promise of a warm welcome, a table awaiting under the dim lights. The digital muse had become a tangible reality, a meal almost tasted.”
Testing and Refinement
The random food place generator, a fragile dream of culinary exploration, must be rigorously tested and refined, much like a chef perfecting a dish, to ensure its functionality and resonance with the user’s yearning for a new experience. This process involves a delicate balance of technical validation and human empathy, acknowledging the fickle nature of taste and the ever-shifting landscape of the digital world.
It’s a journey into the heart of the algorithm, where data dances with desire, and the ultimate goal is to create a truly satisfying experience.
Types of Testing
To ensure the generator functions as intended, various testing methods are essential, each probing a different aspect of its performance. These tests reveal the strengths and weaknesses of the generator, helping to identify areas that need improvement. The following types of testing are necessary:
- Unit Testing: This involves testing individual components of the generator, such as the algorithm that selects restaurants, the filtering mechanisms, and the data retrieval processes. Each unit is isolated and tested to verify that it functions correctly, with specific inputs and expected outputs. For example, a unit test might verify that the distance calculation function correctly determines the distance between a user’s location and a restaurant.
- Integration Testing: This focuses on testing the interaction between different components of the generator. It ensures that these components work together seamlessly, such as the user interface interacting with the data retrieval process. For instance, this test might check if the location data entered by the user is correctly passed to the restaurant search algorithm.
- System Testing: This evaluates the entire generator as a complete system. It assesses the functionality, performance, and security of the generator in a simulated real-world environment. This involves testing all aspects of the generator, from user input to the presentation of results.
- User Acceptance Testing (UAT): This involves real users testing the generator in a realistic environment. It aims to validate that the generator meets the user’s needs and expectations. Users provide feedback on the usability, functionality, and overall experience of the generator.
- Performance Testing: This assesses the generator’s speed, stability, and scalability under various load conditions. This includes load testing (simulating a large number of users), stress testing (testing the generator’s limits), and endurance testing (testing the generator over a prolonged period). For example, performance testing might evaluate how quickly the generator returns results when a large number of users are searching simultaneously.
- Security Testing: This focuses on identifying vulnerabilities in the generator’s security. It ensures that the generator is protected from malicious attacks and data breaches. This includes testing for common vulnerabilities such as SQL injection and cross-site scripting.
Gathering and Utilizing User Feedback
User feedback is the lifeblood of the generator’s evolution, providing invaluable insights into its strengths and weaknesses. Gathering this feedback requires a multifaceted approach, fostering an environment of open communication and continuous improvement. The information gathered should be carefully analyzed and incorporated into the development cycle.
- Feedback Mechanisms: Implement various mechanisms for gathering user feedback, such as:
- In-App Feedback Forms: Provide easy-to-access forms within the generator, allowing users to submit comments, suggestions, and bug reports directly.
- Surveys: Distribute surveys to gather quantitative and qualitative data on user satisfaction, preferences, and pain points.
- User Interviews: Conduct one-on-one interviews with users to gain deeper insights into their experiences and perspectives.
- Social Media Monitoring: Monitor social media channels for mentions of the generator, allowing for the identification of user sentiments and concerns.
- Email Feedback: Provide a dedicated email address for users to send feedback and report issues.
- Analyzing Feedback: Establish a system for analyzing the collected feedback, categorizing it by theme (e.g., usability, accuracy, features) and prioritizing it based on its impact on the user experience. Consider:
- Sentiment Analysis: Use natural language processing (NLP) techniques to analyze the sentiment expressed in user feedback, identifying positive, negative, and neutral opinions.
- Data Visualization: Use charts and graphs to visualize the feedback data, identifying trends and patterns.
- Prioritization: Prioritize feedback based on its frequency, severity, and impact on the user experience. For example, frequently reported bugs or usability issues should be addressed with high priority.
- Implementing Changes: Use the analyzed feedback to inform the development process, making iterative improvements to the generator. This may involve:
- Bug Fixes: Address reported bugs promptly to ensure the generator functions correctly.
- Usability Improvements: Modify the user interface and user experience to improve ease of use and navigation.
- Feature Enhancements: Add new features or modify existing ones based on user requests and preferences.
- Algorithm Refinements: Adjust the underlying algorithms to improve the accuracy and relevance of the generated results.
A/B Testing for Feature Optimization
A/B testing, a dance between two distinct versions of a feature, is a powerful technique for optimizing the generator’s performance. It allows for data-driven decision-making, providing objective evidence of which version resonates more effectively with users. The process involves presenting two versions of a feature (A and B) to different groups of users and measuring their performance based on predefined metrics.
- Defining the Hypothesis: Before starting an A/B test, clearly define the hypothesis to be tested. This hypothesis should state the expected outcome of the test. For example, “Changing the restaurant result display to include the restaurant’s average customer rating will increase click-through rates.”
- Selecting the Metrics: Choose the metrics that will be used to measure the performance of each version. These metrics should be relevant to the goal of the test. For example, click-through rates, conversion rates (e.g., booking a table), time spent on the page, and user engagement are common metrics.
- Creating the Variants: Develop two or more versions of the feature to be tested. Version A is typically the existing version (control), while version B is the modified version (treatment). It’s important to only change one variable at a time to isolate the impact of the change.
- Running the Test: Randomly assign users to each version of the feature. The test should run for a sufficient period to gather enough data to draw statistically significant conclusions. The duration depends on traffic and the expected effect size. A tool like Google Optimize or Optimizely can automate the A/B testing process.
- Analyzing the Results: After the test period, analyze the data to determine which version performed better. Use statistical methods to determine if the difference in performance is statistically significant. A p-value (typically below 0.05) indicates statistical significance, suggesting the observed difference is unlikely due to chance.
- Implementing the Winner: If one version performs significantly better, implement that version for all users. If the results are inconclusive, iterate on the design and run another test.
- Example A/B Test:
Hypothesis: Displaying the restaurant’s average rating will increase click-through rates.
Version A (Control): Restaurant listings show the restaurant’s name, address, and cuisine.
Version B (Treatment): Restaurant listings show the restaurant’s name, address, cuisine, and average customer rating (e.g., 4.5 stars).
Metrics: Click-through rate (percentage of users who click on a restaurant listing).Result: Version B shows a statistically significant increase in click-through rates (e.g., 15% higher than Version A).
Action: Implement Version B for all users.
Examples of Existing Generators
A wistful sigh escapes, mirroring the yearning for culinary discovery. Before our own creation graces the digital landscape, it’s only right to cast a gaze upon the existing stars, the pioneers who have already attempted to quell the hunger for serendipitous dining. These digital muses, each with their own subtle melodies and limitations, offer a glimpse into the possibilities and the challenges that await.
Their successes and failures are like whispers in the wind, guiding us on our journey.
Existing Random Food Place Generators
The world, vast and varied, already holds several digital soothsayers of sustenance. Each generator, a small universe of its own, attempts to distill the chaotic beauty of choice into a simple, clickable moment.
- Yelp’s “Find Food” Feature: This isn’t solely a random generator, but its “Find Food” function, when combined with filters, can yield a degree of randomness. You can specify a location, cuisine type, and price range, and then let Yelp’s algorithm present you with nearby options. The interface, a familiar landscape of maps and listings, is clean and functional, but the lack of true randomness (without extensive filter manipulation) is a minor sorrow.
- Google’s “I’m Feeling Lucky” for Restaurants: A minimalist approach, a whisper of the unknown. Entering “restaurants near me” followed by the “I’m Feeling Lucky” button often leads to a single, randomly selected restaurant. The interface is the stark white canvas of Google’s search, a directness that either charms or leaves one wanting more. Its simplicity can feel like a gentle breeze, but the lack of customization is a quiet ache.
- Random Restaurant Generators (Standalone Websites): Several websites exist solely for this purpose. These often offer more robust filtering options, allowing users to specify cuisine, price, distance, and sometimes even atmosphere (e.g., “romantic,” “family-friendly”). The interfaces vary widely, from simple lists to more visually engaging designs. Some offer integrations with mapping services for directions. These generators, though more comprehensive, can sometimes feel cluttered, a symphony of options that drowns out the quiet beauty of the initial choice.
Comparison of Features and Functionalities
The soul of each generator lies in its capabilities, its ability to shape the user’s experience. A careful comparison reveals both the strengths and the shadows within these digital realms.
- Filtering Options: The ability to refine the search is a critical differentiator. Yelp and dedicated generators often excel here, allowing users to narrow down choices based on cuisine, price, distance, and other preferences. Google’s “I’m Feeling Lucky” offers minimal filtering, embracing a more chaotic approach.
- Data Sources: The quality of the results depends on the underlying data. Yelp and Google leverage their vast databases of user reviews, ratings, and business information. Standalone generators often rely on similar sources, or integrate with third-party APIs.
- User Interface (UI) and User Experience (UX): The visual presentation and ease of use are paramount. Yelp and Google offer familiar, intuitive interfaces. Standalone generators vary, with some prioritizing simplicity and others offering more interactive elements.
- Integration with Other Services: The ability to seamlessly integrate with mapping, reservation, and ordering services enhances the user experience. Yelp and Google offer strong integration, while some standalone generators may have limited capabilities.
- Randomness Algorithm: The core of the generator lies in its algorithm. Some may prioritize proximity, others popularity, and others a truly random selection. The specific algorithm can subtly influence the user’s experience.
Descriptive Illustration of a Generator’s Interface
Let us consider a hypothetical generator, a melancholic echo of possibilities, designed to capture the heart of the user. Imagine an interface, a canvas painted with muted tones of grey and sepia, reminiscent of a vintage photograph.
Imagine the interface with the following components:
- Header: The top section, bearing the generator’s name in a stylized, elegant font, perhaps “Serendipity Suppers.”
- Search Input: A central input field, inviting the user to enter their location, framed by a subtle border.
- Filter Options: Below the input field, a series of elegantly designed filter buttons: “Cuisine Type” (dropdown menu), “Price Range” (sliders), “Distance” (sliders), and “Atmosphere” (buttons like “Romantic,” “Casual,” “Lively”). These buttons are designed with a tactile feel.
- “Generate” Button: A large, inviting button, perhaps with a subtle animation, that says “Find My Supper.”
- Results Area: The main section, where the results appear. A single card, subtly animated, appears after the user clicks “Find My Supper.” The card contains:
- Restaurant Name: Large, prominent text.
- Restaurant Image: A high-quality, evocative image of the restaurant’s exterior or a signature dish.
- Brief Description: A short, poetic description of the restaurant, focusing on its atmosphere and cuisine.
- Address: A clear, concise address.
- Rating: A star rating, if available, displayed in a visually appealing manner.
- “More Info” Button: A button that links to a detailed restaurant profile.
The overall design is clean, minimalist, and aesthetically pleasing, aiming to evoke a sense of calm and anticipation. The use of soft colors and elegant typography creates a sense of sophistication. The focus is on the user experience, with clear navigation and intuitive interactions. The interface is designed to be a portal to culinary adventure, a place where the user can surrender to the joy of the unknown.
Concluding Remarks
From its inception to its practical applications, the random food place generator promises to revolutionize how we approach dining decisions. By harnessing data, algorithms, and user feedback, this tool empowers users to step outside their culinary comfort zones and discover hidden gems. Ultimately, the generator represents a fun and effective solution for those seeking culinary inspiration, promising to add a delightful element of chance to every meal.