Embarking on a flavorful journey, we delve into the fascinating world of the ai food description generator. This innovative technology is revolutionizing how we perceive and interact with food, moving beyond simple lists of ingredients to create truly tantalizing narratives. From its humble beginnings to its current sophisticated capabilities, this system is transforming the way we experience the art of culinary storytelling.
It’s a world where algorithms meet gastronomy, creating a symphony of words that can make your mouth water.
This exploration will unpack the mechanics behind these intelligent systems, unraveling how they process data, generate descriptive text, and adapt to various applications. We’ll explore the benefits, applications, and even the ethical considerations that come with leveraging AI in the realm of food. We will also look into the future, and consider the impact this technology will have on the food industry and the way we savor our meals.
Introduction to AI Food Description Generators
Hello there! Let’s dive into the fascinating world of AI food description generators. These innovative systems are revolutionizing how we perceive and interact with food, offering a glimpse into the future of culinary experiences. Get ready to explore the core of these amazing technologies!These generators are sophisticated tools that use artificial intelligence to create detailed and engaging descriptions of food items.
They analyze various inputs, such as ingredients, preparation methods, and even visual information, to generate text that captures the essence of a dish.
Fundamental Concept of an AI Food Description Generator
At its core, an AI food description generator functions as a sophisticated text generation system. It leverages natural language processing (NLP) and machine learning (ML) to transform data about food into compelling narratives. The system is trained on massive datasets of food-related information, including recipes, culinary terms, and descriptive language. This training enables the AI to understand the relationships between ingredients, cooking techniques, and flavor profiles.
The generator then uses this knowledge to produce descriptions that are both informative and appealing. The ultimate goal is to create descriptions that accurately represent the food while also enticing the reader to try it.
Brief History of the Evolution of These Generators
The journey of AI food description generators has been marked by continuous advancements in AI technology. Early versions were relatively simple, focusing on basic ingredient lists and rudimentary descriptions. However, with the advent of more powerful algorithms and access to larger datasets, these systems have evolved dramatically.
- Early Stages: Initial systems relied on rule-based approaches, where descriptions were generated based on pre-defined rules and templates. This approach was limited in its ability to create nuanced and creative descriptions.
- Mid-Stages: The introduction of machine learning algorithms, particularly neural networks, marked a significant leap forward. These systems could learn from vast amounts of data, enabling them to generate more sophisticated and contextually relevant descriptions.
- Present Day: Current generators utilize advanced NLP techniques, such as transformers, to understand the complexities of language and generate highly detailed and engaging descriptions. They can also incorporate visual information, further enhancing their ability to capture the essence of a dish.
Core Functions and Capabilities of These Systems
AI food description generators possess a wide range of capabilities, enabling them to perform various functions related to food description generation. These functions are driven by the underlying AI models and the data they are trained on.
- Ingredient Analysis: The ability to identify and analyze the ingredients of a dish. This includes understanding the role of each ingredient and its contribution to the overall flavor profile. For example, the generator might recognize that “fresh basil” adds a peppery and aromatic note to a dish.
- Flavor Profile Generation: Creating descriptions of a dish’s flavor profile. This involves using culinary terms and descriptive language to convey the taste and aroma of the food. For instance, it might describe a dish as “savory with a hint of sweetness” or “rich and earthy.”
- Style and Tone Adaptation: Adapting the style and tone of the description to suit different audiences or platforms. For instance, a description for a high-end restaurant menu would be different from a description for a casual food blog.
- Recipe Summarization: Summarizing complex recipes into concise and easy-to-understand descriptions. This can be particularly useful for online recipe platforms and cooking apps.
- Visual Integration: Integrating visual information, such as images of the food, to enhance the descriptions. This can help create a more immersive and engaging experience for the reader. For instance, the AI might describe a “golden-brown roasted chicken” alongside an image of the dish.
How AI Food Description Generators Work
Hello there! Let’s delve into the fascinating mechanics behind AI food description generators. These systems are not just magic; they are sophisticated applications of artificial intelligence, carefully designed to transform raw data into compelling and appetizing descriptions. Understanding the inner workings of these generators provides valuable insight into how they create such engaging content.
Data Input and Processing
The process begins with data input and its subsequent processing. The quality and variety of the input data are crucial to the generator’s performance.The data used by these generators typically encompasses:
- Food Images: The AI analyzes visual data, such as images of the food item. This helps in identifying key features like color, shape, and texture. For example, the system might note the glistening glaze on a glazed donut or the vibrant colors of a fruit salad.
- Textual Descriptions: Existing descriptions of food items, including ingredient lists, recipes, and reviews, provide the AI with a wealth of information about flavors, aromas, and culinary techniques. This includes details from various sources, such as restaurant menus, online food blogs, and culinary databases.
- Ingredient Information: Data on individual ingredients, including their flavor profiles, origins, and nutritional information, is fed into the system. This allows the AI to understand the building blocks of the dish.
- Nutritional Data: Information on the nutritional content of foods, such as calories, macronutrients, and micronutrients, can also be integrated.
- User Reviews and Ratings: Feedback from users helps the AI understand what people enjoy about a particular dish.
The processing stage involves several steps:
- Data Cleaning: This involves removing irrelevant information, correcting errors, and standardizing the data format.
- Feature Extraction: The AI extracts relevant features from the data, such as the color of a sauce or the presence of certain ingredients.
- Data Encoding: Text and image data are converted into numerical representations that the AI can understand. This is often done using techniques like word embeddings for text and convolutional neural networks for images.
- Data Integration: All the different types of data are combined to create a comprehensive understanding of the food item.
Algorithms Used in These Generators
AI food description generators leverage a variety of algorithms to produce compelling text. These algorithms are trained on large datasets to learn patterns and relationships within the data.Common algorithms include:
- Natural Language Processing (NLP) models: These models are used to understand and generate human language. They can analyze the structure of sentences, identify the key features of a dish, and generate descriptive text.
- Convolutional Neural Networks (CNNs): CNNs are used to analyze images of food, extracting visual features such as color, texture, and shape. These features are then used to inform the text generation process.
- Recurrent Neural Networks (RNNs) and Transformers: RNNs, especially Long Short-Term Memory (LSTM) networks, and more recently, Transformer models, are particularly effective at processing sequential data, such as text. They are used to generate coherent and contextually relevant descriptions by considering the relationships between words and phrases.
- Generative Adversarial Networks (GANs): Although less common, GANs can be used to generate realistic food descriptions by pitting two neural networks against each other: a generator that creates descriptions and a discriminator that tries to identify fake descriptions.
These algorithms work together to analyze the data, identify key features, and generate descriptive text that captures the essence of the food item.
Generating Descriptive Text
The final step involves the AI system crafting the actual descriptive text. This process relies on the information gathered during data input and processing, as well as the capabilities of the algorithms employed.The process of generating descriptive text typically involves:
- Feature Identification: The AI identifies the key features of the food item, such as its ingredients, flavors, and visual appearance.
- Concept Generation: The AI generates a set of concepts or ideas related to the food item, such as “savory,” “sweet,” “creamy,” or “crispy.”
- Sentence Construction: The AI uses the identified features and concepts to construct sentences that describe the food item. This may involve using pre-defined templates or generating text from scratch.
- Style and Tone Adaptation: The AI can be trained to adapt its writing style and tone based on the target audience or the desired effect. For example, it might use more formal language for a fine-dining restaurant or more casual language for a fast-food menu.
- Output Generation: The system generates the final descriptive text, which is then presented to the user.
Here’s a table summarizing the steps involved:
Step | Description | Example | Algorithms Involved |
---|---|---|---|
Data Input | Gathering of information, including images, text, and nutritional data. | Collecting photos of a pizza, ingredient lists, and customer reviews. | N/A |
Data Processing | Cleaning, encoding, and integrating the input data. | Removing errors from ingredient lists and converting images into numerical data. | NLP, CNNs |
Feature Extraction | Identifying key characteristics from the data. | Determining the color, texture, and ingredients of the pizza. | CNNs, NLP |
Text Generation | Constructing descriptive sentences. | Creating text that describes the pizza as having a crispy crust, savory tomato sauce, and melted mozzarella cheese. | RNNs, Transformers |
Benefits of Using AI for Food Descriptions
Hello there! We’ve explored the inner workings of AI food description generators. Now, let’s dive into the exciting advantages these tools bring to the table. From boosting efficiency to ensuring consistency, AI is revolutionizing how we describe and present food.
Efficiency and Cost-Effectiveness of AI
One of the most compelling benefits of using AI for food descriptions is the significant boost in efficiency and the associated cost savings. Manual creation of food descriptions is time-consuming, requiring skilled writers, photographers, and editors. AI automates much of this process, drastically reducing the time and resources needed.AI excels at quickly generating descriptions, which allows businesses to update menus, online platforms, and marketing materials much faster than manual methods permit.
This speed translates directly into cost savings, as it minimizes the need for extensive human labor. Moreover, AI can be trained on vast datasets, enabling it to create a large volume of descriptions simultaneously, something human writers would struggle to match. This accelerated output is especially beneficial for businesses with extensive menus or frequently changing offerings.
Accuracy and Consistency Improvement with AI
AI food description generators are particularly effective at improving the accuracy and consistency of food descriptions. Human writers can be prone to errors, inconsistencies, and subjective biases, leading to variations in descriptions across a menu or platform. AI, on the other hand, can be programmed to adhere to specific guidelines and styles, ensuring that descriptions are consistently accurate and follow a pre-defined format.AI’s ability to analyze data and learn patterns allows it to identify and correct inaccuracies, such as misspellings or incorrect ingredient listings.
The consistent application of a chosen tone and style helps to maintain a cohesive brand voice across all food descriptions. This consistency is crucial for building trust with customers and ensuring a positive user experience. For instance, imagine an online food delivery platform. If the descriptions of the same dish vary in detail and tone across different restaurants, customers may find it difficult to make informed choices.
AI can help standardize the descriptions, leading to a more uniform and reliable experience.
Advantages of AI Food Description Generators
Here’s a quick overview of the key benefits:
Advantage | Description | Impact |
---|---|---|
Increased Efficiency | AI generates descriptions much faster than manual methods. | Saves time and reduces labor costs, enabling faster updates and content creation. |
Cost Reduction | Automates the creation process, minimizing the need for human writers and editors. | Reduces expenses associated with content creation, leading to higher profit margins. |
Improved Accuracy | AI can analyze data to identify and correct inaccuracies, such as misspellings or incorrect ingredient listings. | Ensures descriptions are factual and reliable, building customer trust and satisfaction. |
Enhanced Consistency | AI adheres to pre-defined guidelines and styles, ensuring uniform descriptions across all platforms. | Maintains a cohesive brand voice and improves the overall user experience. |
Applications of AI Food Descriptions
AI food description generators are transforming how we experience and interact with food information. These tools are not just novelties; they are powerful assets being implemented across a variety of industries, enhancing user experiences and streamlining operations. The applications are diverse, spanning from online platforms to physical menus, and their impact is continually growing.
Industries Utilizing AI Food Description Generators
Several industries are actively leveraging AI food description generators to improve their services and offerings. These generators provide a competitive edge by automating content creation and delivering engaging and informative descriptions.
- Food Retailers: Grocery stores and food delivery services utilize AI to create detailed product descriptions for online listings, enhancing searchability and customer engagement. For instance, a supermarket might use AI to generate descriptions for a new line of artisanal cheeses, highlighting flavor profiles, origin, and pairing suggestions.
- Restaurant Industry: Restaurants, from fast-food chains to fine dining establishments, employ AI to craft compelling menu descriptions that entice customers. AI can generate descriptions tailored to specific cuisines or dietary needs, improving menu accessibility and appeal.
- Food Blogging and Media: Food bloggers and culinary publications use AI to create unique and -friendly content for recipes, restaurant reviews, and food-related articles. AI can assist in generating diverse content, including variations of descriptions and ingredient information.
- Food Tech Companies: Companies specializing in food technology and innovation use AI to develop new applications for food-related content, such as personalized recipe recommendations and dietary analysis tools.
Use in Online Food Ordering Platforms
Online food ordering platforms benefit significantly from AI-generated food descriptions. These platforms require extensive and engaging content to attract and inform customers.
- Enhanced Product Descriptions: AI can generate detailed and descriptive information for each menu item, including ingredients, preparation methods, and flavor profiles. This allows customers to make informed decisions, increasing the likelihood of a purchase.
- Improved Search and Discovery: AI-powered descriptions improve the searchability of menu items, allowing customers to find what they are looking for quickly. Platforms can use AI to suggest similar items based on a customer’s preferences.
- Personalized Recommendations: AI can analyze customer data to provide personalized food recommendations, based on past orders, dietary restrictions, and preferences. This enhances the user experience and increases customer satisfaction.
- Automated Content Updates: AI can automatically update menu descriptions based on changes in ingredients, pricing, or availability, ensuring the information is always current and accurate.
Role in Restaurant Menus and Food Blogs
AI food descriptions play a crucial role in enhancing the appeal and effectiveness of restaurant menus and food blogs. These tools allow for the creation of more engaging and informative content.
- Compelling Menu Descriptions: Restaurants can use AI to craft menu descriptions that highlight the unique features and flavors of each dish, enticing customers to try new items. This can include information about the chef’s inspiration, the origin of ingredients, and recommended pairings.
- Optimization: Food bloggers and restaurants can use AI to optimize their content for search engines, ensuring that their menus and recipes are easily found by potential customers. This includes the use of relevant s and phrases in descriptions.
- Content Variety: AI can assist in generating different variations of descriptions, allowing for A/B testing to determine which descriptions perform best. This can help optimize the content for different audiences and platforms.
- Multilingual Support: AI can translate menu descriptions into multiple languages, making them accessible to a wider audience. This is particularly useful for restaurants in tourist areas or those with international clientele.
Table: Applications of AI Food Description Generators
This table illustrates the different applications of AI food description generators across various industries and platforms, highlighting their impact on content creation, user experience, and business operations.
Industry/Platform | Application | Benefit | Example |
---|---|---|---|
Online Food Ordering Platforms | Detailed Product Descriptions | Increased Customer Engagement and Sales | Generating descriptions for menu items, including ingredients, preparation methods, and flavor profiles. |
Restaurant Menus | Compelling Menu Descriptions | Enhanced Customer Appeal and Order Volume | Crafting descriptions that highlight unique features and flavors, including the chef’s inspiration and origin of ingredients. |
Food Blogs | Optimization and Content Variety | Improved Search Rankings and Audience Reach | Optimizing recipe descriptions with relevant s and generating different variations for A/B testing. |
Food Retailers | Product Listings and Enhanced Searchability | Improved Customer Discovery and Purchase Decisions | Creating detailed descriptions for grocery items, highlighting flavor profiles, origin, and pairing suggestions. |
Data and Training: Fueling the AI
The magic behind AI food description generators lies in the massive amounts of data they’re fed. This data is the fuel that powers these models, enabling them to learn and understand the nuances of food, from its appearance and aroma to its taste and texture. The quality and quantity of this data directly impact the AI’s ability to generate accurate, engaging, and creative descriptions.
Importance of Training Data
Training data is the cornerstone of any successful AI model. Without it, the AI has nothing to learn from, making it impossible to generate meaningful or relevant outputs. The training process involves feeding the AI a vast collection of examples, allowing it to identify patterns, correlations, and relationships within the data. This learning process is crucial for enabling the AI to understand the complexities of food and language.
The more comprehensive and diverse the training data, the better the AI becomes at generating high-quality food descriptions.
Types of Data Used to Train AI Models
AI food description generators utilize a variety of data types to learn about food. These data sources, when combined, provide a holistic understanding of the subject matter, leading to more detailed and accurate descriptions.* Textual Data: This includes recipes, food blogs, restaurant menus, and product descriptions. This data provides the AI with information about ingredients, preparation methods, flavor profiles, and culinary styles.
Image Data
Images of food are essential for teaching the AI about visual characteristics. The AI learns to associate visual features like color, shape, and arrangement with specific dishes and ingredients. For example, an AI model might learn to identify a perfectly seared steak based on its color and the presence of grill marks.
Sensory Data
This type of data can include descriptions of taste, smell, and texture. It might involve data from professional food critics, flavor profiles, or sensory analysis reports.
Nutritional Data
Information on nutritional content, such as calories, macronutrients, and micronutrients, can be incorporated to provide more comprehensive descriptions.
Impact of Data Quality on Output
The quality of the training data has a profound impact on the AI’s ability to generate accurate and compelling food descriptions. Poor-quality data can lead to inaccurate, nonsensical, or even misleading outputs. For example, if the training data contains inconsistent or incorrect ingredient information, the AI might generate a description that lists the wrong ingredients or combines flavors that don’t complement each other.
Conversely, high-quality data allows the AI to generate descriptions that are not only accurate but also creative and engaging.Let’s consider a scenario where the AI is trained on data about different types of coffee.* Poor Data Quality Example: If the data contains typos, inconsistent terminology (e.g., “latte” sometimes referred to as “caffe latte” and sometimes not), or incorrect information about brewing methods, the AI might generate descriptions that are confusing or inaccurate.
The AI might, for example, describe a “flat white” as having whipped cream, which is incorrect.
High Data Quality Example
With clean, well-labeled data that includes details on origin, roasting process, brewing methods, and tasting notes, the AI can generate nuanced descriptions, such as “This single-origin Ethiopian Yirgacheffe boasts bright acidity, floral aromas, and a clean finish, brewed using a pour-over method to highlight its delicate flavors.”
Sources of Data Used for Training
AI food description generators draw upon a wide array of data sources to build their knowledge base. These sources provide the diverse information needed to create comprehensive and accurate descriptions.* Online Recipe Databases: Websites like Allrecipes, Food.com, and BBC Good Food offer extensive collections of recipes, providing valuable information on ingredients, preparation methods, and cultural variations.
Food Blogs and Culinary Websites
Blogs and websites dedicated to food and cooking provide rich textual data, including detailed descriptions of dishes, ingredient pairings, and culinary techniques.
Restaurant Menus
Menus from restaurants worldwide provide valuable information on dish names, ingredients, and pricing.
Product Descriptions
Descriptions of food products, such as those found on grocery store websites or in product catalogs, offer insights into ingredients, nutritional information, and preparation instructions.
Books and Publications
Cookbooks, food magazines, and scientific publications provide expert knowledge on food science, culinary techniques, and flavor profiles.
User-Generated Content
Reviews, ratings, and comments from users on food-related platforms provide insights into consumer preferences and perceptions of different dishes.
Accuracy and Limitations
While AI food description generators offer exciting possibilities, it’s crucial to acknowledge their current limitations and potential inaccuracies. The quest for perfect descriptions is ongoing, and understanding these challenges is key to using these tools effectively. The goal is to leverage their strengths while being mindful of their weaknesses.
Challenges in Achieving High Accuracy
Achieving consistently high accuracy in AI-generated food descriptions is a complex undertaking. Several factors contribute to the difficulty. The diversity of food itself, the nuances of culinary language, and the subjective nature of taste all pose significant hurdles. The following paragraphs provide detailed explanations of the issues.The variability in food preparation methods across different cultures and regions adds to the complexity.
A single dish can have countless variations. For example, a “pizza” can range from a simple Margherita to a complex gourmet creation with various toppings and crust types. The AI must learn and differentiate between these variations, which requires extensive and diverse training data.Furthermore, the subjective nature of taste perception makes accuracy challenging. What one person considers “delicious” or “perfectly seasoned,” another might find bland or overpowering.
Capturing these individual preferences and incorporating them into a universally appealing description is a significant hurdle for AI models. The use of specific language and terminology is also very important.
Potential Limitations of AI-Generated Food Descriptions
AI-generated descriptions, despite advancements, have inherent limitations. Understanding these limitations is crucial for responsible usage.Here are some of the potential drawbacks:
- Lack of Contextual Understanding: AI may struggle to grasp the full context of a dish, including its cultural significance, historical background, or the chef’s intentions. For example, it might describe a traditional dish based on ingredients without understanding its cultural importance.
- Inability to Capture Nuance and Subjectivity: AI often struggles with the subtle nuances of taste, texture, and aroma. It might provide a generic description that fails to capture the unique qualities that make a dish special.
- Over-Reliance on Training Data: The accuracy of the descriptions heavily depends on the quality and diversity of the training data. If the data is biased or incomplete, the AI will likely produce inaccurate or misleading descriptions. For example, if the training data primarily features Western cuisine, the AI might struggle to accurately describe dishes from other culinary traditions.
- Difficulty with Complex or Novel Dishes: AI may find it challenging to describe dishes that are highly complex, use unusual ingredients, or are newly created. It may not have sufficient data to accurately capture their characteristics.
- Potential for Generating Nonsensical or Inconsistent Descriptions: In some cases, AI can generate descriptions that are grammatically correct but lack coherence or make illogical statements. For example, the AI might claim that a dish contains ingredients that are not usually associated with it.
- Ethical Concerns: There are ethical concerns about the potential for AI to perpetuate biases in food descriptions. If the training data reflects existing biases, the AI may reinforce them, leading to unfair or inaccurate representations of certain cuisines or ingredients.
Examples of Common Errors or Inaccuracies
AI food description generators can make a variety of errors. These errors stem from the challenges in understanding food’s complexity and the nuances of language. Here are some examples:* Incorrect Ingredient Identification: The AI might misidentify an ingredient, leading to an inaccurate description. For example, it might describe a dish as containing “cilantro” when it actually uses “parsley.” This can lead to misunderstandings, especially for people with allergies or strong dislikes.
Exaggerated or Misleading Sensory Descriptions
AI may generate descriptions that are overly enthusiastic or use inaccurate sensory details. For example, it might describe a dish as “bursting with flavor” when the flavor profile is actually quite subtle. Or, it could state that a dish is “crispy” when the texture is actually soft.
Inaccurate Cultural References
The AI might make incorrect cultural references or fail to recognize the origin of a dish. For example, it might mistakenly associate a dish with a country or region that is not its origin.
Inconsistent Terminology
The AI might use inconsistent terminology to describe the same dish or ingredient, leading to confusion. For example, it might refer to “eggplant” in one sentence and “aubergine” in another.
Failure to Differentiate Between Similar Dishes
The AI might struggle to distinguish between similar dishes, leading to inaccurate descriptions. For example, it might describe a “pasta carbonara” as if it were a “pasta Alfredo.”
User Experience and Engagement
AI-powered food description generators are transforming the way customers interact with food businesses online and in-store. The effectiveness of these descriptions significantly impacts customer engagement, influencing purchasing decisions and overall satisfaction. Understanding how to optimize these descriptions for user experience is crucial for any business looking to thrive in the competitive food industry.
How AI Descriptions Affect Customer Engagement
AI-generated food descriptions influence customer engagement through several key mechanisms. These descriptions often create a more immersive and informative experience, leading to increased interest and interaction.
- Enhanced Information: AI can provide detailed information about ingredients, preparation methods, and nutritional facts, allowing customers to make informed choices. This level of detail is particularly beneficial for those with dietary restrictions or specific preferences.
- Improved Accessibility: AI can translate descriptions into multiple languages, making menus and product information accessible to a wider audience. This inclusivity enhances the user experience for diverse customer bases.
- Personalized Recommendations: AI algorithms can analyze customer preferences and browsing history to generate tailored food descriptions and recommendations. This personalization makes the customer feel understood and valued, boosting engagement.
- Increased Visual Appeal: AI can be used to generate descriptions that are more evocative and engaging, using descriptive language that appeals to the senses. This can lead to a higher click-through rate and increased time spent on product pages.
Methods for Optimizing Descriptions for Better User Experience
Optimizing AI-generated food descriptions involves a multifaceted approach, focusing on clarity, accuracy, and emotional appeal. These strategies ensure that the descriptions resonate with customers and drive positive engagement.
- Prioritize Clarity and Accuracy: Ensure descriptions are easy to understand and factually correct. Avoid overly complex language or misleading information. For example, if a dish is spicy, clearly state the level of spiciness.
- Incorporate Sensory Details: Use descriptive language that appeals to the senses – sight, smell, taste, and texture. Instead of simply stating “chocolate cake,” describe it as “a rich, decadent chocolate cake with a moist crumb, a deep chocolate aroma, and a smooth, velvety frosting.”
- Highlight Unique Selling Points: Emphasize what makes a dish special. Is it locally sourced ingredients? A unique preparation method? A family recipe? These details add value and attract customers.
- Use s Effectively: Integrate relevant s that customers might use when searching for food items. This improves search engine optimization () and increases the visibility of your descriptions.
- Maintain Consistency: Ensure that the tone and style of descriptions are consistent across all platforms. This creates a cohesive brand identity and a more seamless user experience.
Examples of Successful and Unsuccessful Descriptions
The effectiveness of a food description can vary significantly. Examining successful and unsuccessful examples provides valuable insights into best practices and common pitfalls.
- Successful Example: “Our signature Margherita pizza features a thin, crispy crust topped with San Marzano tomatoes, fresh mozzarella, fragrant basil, and a drizzle of extra virgin olive oil. Baked to perfection in our wood-fired oven, it offers a symphony of flavors with every bite.” This description is detailed, sensory-rich, and highlights the pizza’s key features.
- Unsuccessful Example: “Pizza with toppings.” This description is vague, uninformative, and fails to engage the customer. It lacks any details about ingredients, preparation, or flavor profile.
Comparison of Food Description Styles
Different styles of food descriptions can be employed to appeal to various audiences. The best approach often depends on the target demographic and the nature of the food item.
Style | Description Example | Pros | Cons |
---|---|---|---|
Descriptive | “Creamy avocado slices nestled on a bed of mixed greens, drizzled with a zesty lime vinaigrette, and sprinkled with toasted pumpkin seeds.” | Evokes sensory details; creates a strong emotional connection; ideal for highlighting ingredients and preparation. | Can be time-consuming to create; requires strong writing skills; may be perceived as overly verbose. |
Informative | “Gluten-free quinoa salad with grilled chicken, black beans, corn, and a light cilantro-lime dressing. Contains 350 calories, 20g protein, and 10g fat.” | Provides essential nutritional information; appeals to health-conscious customers; suitable for quick reference. | May lack emotional appeal; can be less engaging; may not highlight the unique qualities of the dish. |
Benefit-Oriented | “Indulge in our rich chocolate fudge brownie, made with premium cocoa and guaranteed to satisfy your sweet cravings.” | Focuses on the customer’s experience; emphasizes the value proposition; highlights the benefits of the dish. | May sound overly promotional; can be less informative about the actual ingredients; relies on subjective claims. |
Future Trends and Developments
The field of AI food description generation is dynamic, with continuous advancements promising to revolutionize how we perceive and interact with food. These future trends are poised to significantly impact the food industry, from restaurant menus to online grocery shopping experiences. We can anticipate even more sophisticated and nuanced descriptions, reflecting the evolving tastes and preferences of consumers.
Browse the implementation of foods to eat on zepbound in real-world situations to understand its applications.
Emerging Trends in AI Food Description Generation
Several key trends are emerging, shaping the future of AI-powered food descriptions. These advancements promise to create richer, more engaging, and informative experiences for consumers.* Enhanced Sensory Descriptions: AI will increasingly incorporate more detailed sensory information, moving beyond basic taste and texture descriptions. Expect AI to generate descriptions that evoke smells, sounds (e.g., the sizzle of a steak), and even the visual appeal of a dish with greater precision.
For example, an AI might describe a coffee’s aroma as “hints of dark chocolate and toasted nuts, with a subtle floral undertone.”* Personalized Food Descriptions: AI will leverage user data, including dietary restrictions, allergies, and flavor preferences, to generate personalized food descriptions. This could include highlighting ingredients suitable for specific diets (e.g., gluten-free, vegan) or suggesting alternative dishes based on a user’s past choices.
This level of personalization is already being explored by companies like ChowNow, which uses AI to suggest dishes based on a user’s order history and dietary needs.* Integration with Image Recognition: AI will seamlessly integrate image recognition technology to analyze food images and generate descriptions that correlate visual details with textual information. For example, the AI might identify the type of bread used in a sandwich, the specific herbs sprinkled on a dish, or the cooking method employed, further enriching the description.* Multilingual Capabilities: As globalization continues, AI-powered food descriptions will expand their multilingual capabilities, enabling restaurants and food businesses to cater to a diverse customer base.
This will involve accurate translations of descriptions and the ability to tailor descriptions to cultural nuances.* Real-time Data Integration: AI will leverage real-time data, such as ingredient availability, seasonal changes, and chef specials, to generate dynamic and up-to-date food descriptions. This will ensure that descriptions are always accurate and reflect the current menu offerings.
Future Improvements and Innovations in this Field
The future holds significant improvements and innovations for AI food description generation. These advancements will focus on accuracy, detail, and user engagement.* Improved Accuracy and Nuance: Future AI models will be trained on even larger and more diverse datasets, leading to greater accuracy in describing food characteristics. This will include capturing subtle flavor profiles, textures, and preparation methods.* Advanced Emotional Analysis: AI could be developed to analyze food descriptions to understand and convey the emotional impact of a dish.
For example, it might describe a comfort food dish as “warm and nostalgic,” or a spicy dish as “bold and invigorating.”* Interactive Food Experiences: AI could be used to create interactive food experiences, such as virtual cooking classes or personalized recipe recommendations. This would involve using AI to provide step-by-step instructions and guidance to users, making cooking more accessible and enjoyable.* Integration with Augmented Reality (AR): AR technology could be combined with AI-generated food descriptions to provide users with a more immersive and informative experience.
Users could point their smartphone cameras at a dish and receive detailed information about its ingredients, nutritional value, and preparation method.* Greater Emphasis on Sustainability and Ethical Sourcing: AI models will be trained to highlight the sustainability and ethical sourcing of ingredients. This would involve providing information about where ingredients are sourced from, how they are produced, and their environmental impact.
Potential Impact of These Developments on the Food Industry
These advancements will have a profound impact on the food industry, transforming how businesses operate and how consumers interact with food.* Enhanced Menu Creation: Restaurants can use AI to create more descriptive and appealing menus, attracting more customers and increasing sales. The AI could suggest menu pairings, create descriptions that cater to specific dietary needs, and automatically update menus based on ingredient availability.* Improved Online Food Ordering: Online food ordering platforms can leverage AI to provide more accurate and informative food descriptions, making it easier for customers to find what they want and make informed decisions.
This will also reduce the number of order errors and improve customer satisfaction.* Personalized Food Recommendations: AI can provide personalized food recommendations based on user preferences, dietary restrictions, and past orders. This will improve the customer experience and encourage repeat business.* Streamlined Food Production: AI can be used to optimize food production processes, from ingredient sourcing to recipe development.
This will lead to increased efficiency, reduced waste, and improved food quality.* Enhanced Food Education: AI can be used to educate consumers about food, including its ingredients, nutritional value, and preparation methods. This will empower consumers to make healthier choices and become more knowledgeable about food.
Ethical Considerations
As we delve into the realm of AI food description generators, it’s crucial to address the ethical dimensions of this technology. While these tools offer exciting possibilities, we must be mindful of the potential for misuse and the importance of responsible development and deployment. Ensuring fairness, transparency, and accountability is paramount to prevent unintended consequences and build trust in this emerging field.
Bias in Generated Text
AI models learn from the data they are trained on. This data can inadvertently contain biases reflecting societal stereotypes or historical inequalities. If not carefully addressed, these biases can be amplified and perpetuated in the generated food descriptions.
- Food Preferences and Cultural Representation: AI might be trained on data that overrepresents certain cuisines or dietary preferences, leading to less accurate or even biased descriptions of less-represented foods. For example, an AI trained primarily on Western cuisine data might struggle to accurately describe the nuances of a complex dish from Southeast Asia.
- Stereotyping and Discrimination: Biases in the training data can lead to descriptions that reinforce stereotypes about certain demographics or food cultures. This could manifest in subtle ways, such as associating specific foods with particular ethnicities or social groups in a way that is inaccurate or offensive.
- Ingredient Availability and Accessibility: Descriptions generated might implicitly assume access to specific ingredients, which might not be readily available or affordable for everyone. This can exclude or misrepresent dishes that rely on locally sourced or less common ingredients.
Ensuring Fairness and Transparency
Mitigating bias and promoting ethical AI requires a multi-faceted approach. Developers and users of AI food description generators have a responsibility to implement measures that foster fairness and transparency.
- Data Auditing and Curation: Rigorous auditing of the training data is crucial to identify and mitigate biases. This includes examining the sources of the data, the representation of different food types and cultures, and the potential for stereotypes. Data curation involves actively removing or correcting biased data and supplementing it with more diverse and representative information.
- Bias Detection and Mitigation Techniques: Employing various techniques to detect and mitigate bias within the AI model itself. This can include using fairness-aware algorithms, debiasing techniques, and regular model evaluations to identify and address potential biases in the generated text.
- Transparency and Explainability: Striving for transparency in how the AI generates descriptions. This involves making the model’s decision-making process more understandable, such as providing information about the data sources, the algorithms used, and the limitations of the model.
- User Feedback and Iteration: Collecting user feedback on the generated descriptions and using this feedback to refine the model and address any biases or inaccuracies. This iterative process ensures that the AI continues to improve and better reflects the diversity of the culinary world.
Ethical Considerations Summary
Ethical Concern | Description | Mitigation Strategies |
---|---|---|
Bias in Data | AI models trained on biased data may perpetuate stereotypes, misrepresent cuisines, and exclude certain demographics. | Data auditing, bias detection and removal, and diversification of training data. |
Lack of Transparency | Opacity in how descriptions are generated can lead to a lack of trust and make it difficult to identify and correct errors or biases. | Model explainability, clear documentation, and user feedback mechanisms. |
Accessibility and Fairness | Generated descriptions might implicitly assume access to specific ingredients or cater to limited dietary preferences, potentially excluding certain consumers. | Inclusive language, diverse descriptions, and consideration of ingredient availability and cultural context. |
Building Your Own AI Food Description System (Simplified)
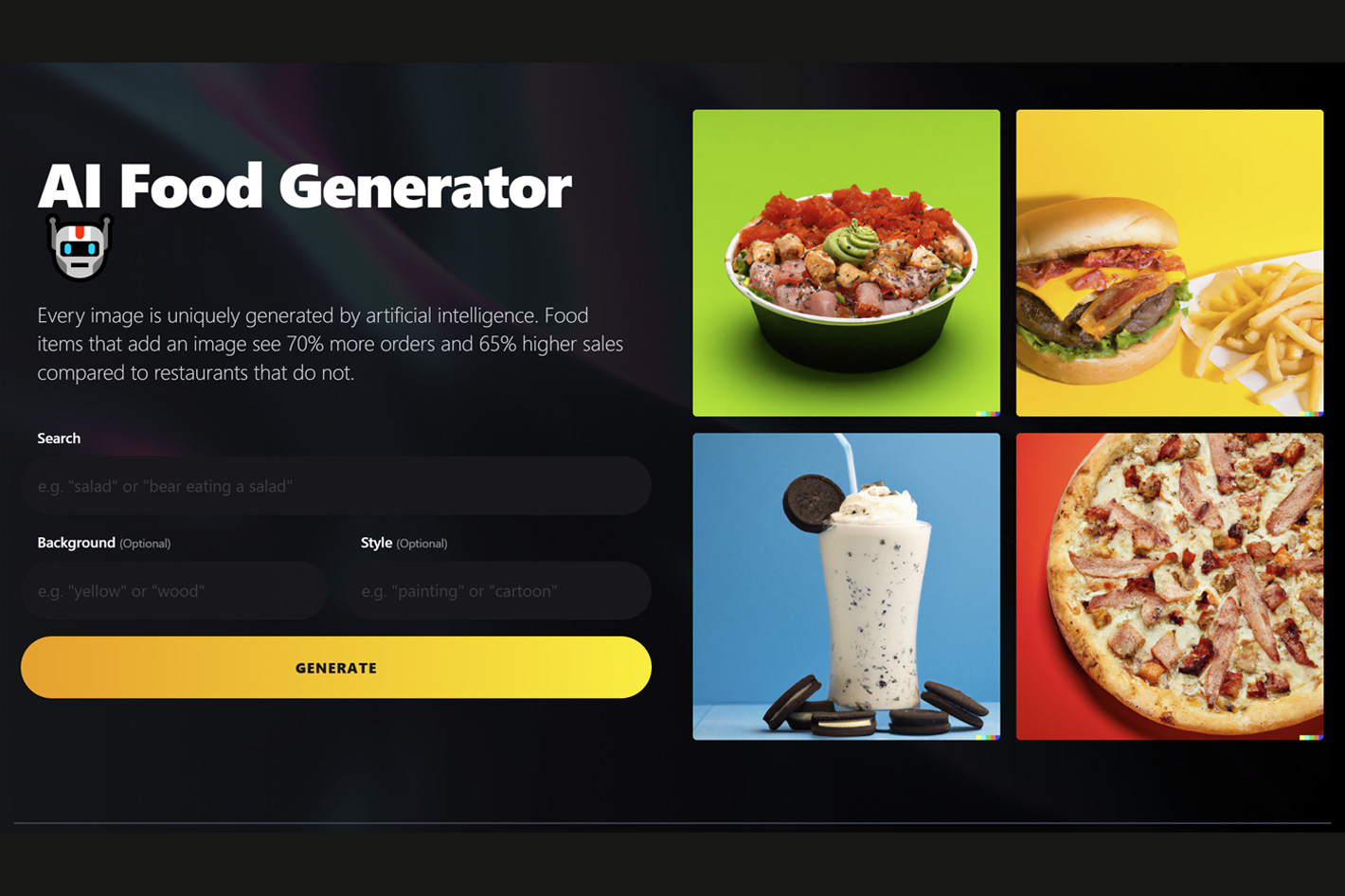
Creating your own AI food description system might seem daunting, but with a simplified approach, it’s surprisingly achievable. This section provides a practical guide to building a basic system, focusing on core concepts and readily available tools. We’ll break down the process into manageable steps, enabling you to generate food descriptions with your own customized model.
Data Collection and Preparation
Data is the fuel that powers any AI system. For food descriptions, this means gathering a substantial dataset of food items and their corresponding descriptions. The quality and diversity of your data directly impact the performance of your AI.To collect and prepare data, consider these strategies:
- Data Sources: Start by exploring publicly available datasets. Kaggle, for instance, hosts datasets related to food images and descriptions. Websites like Allrecipes.com and food.com offer a wealth of recipe data that can be scraped (with proper permissions and ethical considerations). Restaurants’ online menus can also provide valuable descriptions.
- Data Scraping: Use web scraping tools like Beautiful Soup (Python) or Octoparse to extract data from websites. Be mindful of robots.txt files and respect website usage policies.
- Manual Data Entry: Supplement scraped data with manually created descriptions, especially for specific culinary styles or cuisines. This adds valuable nuance.
- Data Cleaning: This is a critical step. Clean your data by removing irrelevant characters, correcting spelling errors, and standardizing formatting. Consider using natural language processing (NLP) libraries like NLTK or spaCy to tokenize, stem, and lemmatize the text data.
- Data Formatting: Organize your data into a structured format, such as a CSV file or a database. Each row should represent a food item, with columns for the food item name, a description, and potentially other relevant features like ingredients, cooking style, or nutritional information.
- Data Augmentation: Increase the size and diversity of your dataset. This can involve paraphrasing existing descriptions or creating variations of descriptions based on ingredients and cooking methods.
Tools and Technologies Needed
Building an AI food description system requires a combination of tools and technologies. Fortunately, many open-source options are available, making it accessible even for those with limited coding experience.The core components include:
- Programming Language: Python is the dominant language for AI development due to its extensive libraries and community support.
- NLP Libraries: Libraries like NLTK (Natural Language Toolkit) and spaCy are essential for text processing tasks such as tokenization, stemming, and part-of-speech tagging. These help in understanding and manipulating the text data.
- Machine Learning Frameworks: TensorFlow and PyTorch are the leading frameworks for building and training machine learning models. They provide the necessary tools for creating neural networks.
- Text Generation Models: Consider using pre-trained language models like GPT-2 or GPT-3 (via APIs) for generating text. Fine-tuning these models on your food description dataset can yield excellent results. Alternatively, you can build your own recurrent neural network (RNN) or transformer-based model from scratch.
- Data Storage: Use CSV files, databases (like SQLite or PostgreSQL), or cloud storage (like AWS S3 or Google Cloud Storage) to store your data.
- Development Environment: Use an Integrated Development Environment (IDE) like VS Code or PyCharm to write and debug your code. Jupyter Notebooks are also useful for interactive data exploration and model prototyping.
Steps Involved in Creating a Basic AI Food Description System
Here’s a simplified, step-by-step guide to building your own basic AI food description system:
- Data Collection and Preparation: Gather and clean your data as described above.
- Model Selection: Choose a suitable model architecture. For a simplified system, consider fine-tuning a pre-trained language model (e.g., GPT-2) or training a simple RNN.
- Data Preprocessing: Prepare your data for the model. This may involve tokenizing the text, creating word embeddings, and splitting the data into training and testing sets.
- Model Training: Train your chosen model on your prepared dataset. This involves feeding the data to the model and adjusting its parameters to minimize errors. Monitor the training process using metrics like loss and accuracy.
- Model Evaluation: Evaluate the performance of your trained model on a held-out test set. Use metrics like perplexity and BLEU score to assess the quality of the generated descriptions.
- Description Generation: Use your trained model to generate new food descriptions. Provide the model with a prompt (e.g., the name of a food item) and let it generate the description.
- Refinement and Iteration: Review the generated descriptions and identify areas for improvement. This might involve collecting more data, fine-tuning the model further, or adjusting the model’s parameters. Repeat the process to enhance the system’s performance.
For example, a simplified implementation might involve using the Hugging Face Transformers library in Python to fine-tune a pre-trained GPT-2 model. You would load your dataset, tokenize the text, and then train the model on your data. The model would then be able to generate descriptions based on input prompts.
Closing Notes
In conclusion, the ai food description generator represents a significant advancement in how we communicate about food. From streamlining operations to enriching customer experiences, the potential of this technology is vast and ever-evolving. As we continue to refine these systems and address their limitations, the future promises even more compelling and personalized culinary narratives. It’s a journey that blends technology with the timeless art of cooking, creating a feast for the senses, and inviting us to explore the delightful possibilities of the food we love.