Alright foodies, let’s talk fast food! As the fast food chooser, this isn’t just about craving a burger; it’s about making smart choices in a world overflowing with options. Think of it as your personal food concierge, guiding you through the drive-thru jungle. We’re diving deep, exploring how this tool can revolutionize your quick-bite game, from understanding the core concept to crafting personalized recommendations.
We’ll explore user personas, imagine the essential features, and dive into the technical aspects of building such a tool. Imagine being able to compare nutrition facts on the fly, find the closest options, and even discover hidden gems you never knew existed. This is all about making informed decisions, even when time is of the essence. Get ready to level up your fast-food experience!
Defining the “Fast Food Chooser” Concept
The “Fast Food Chooser” is a digital application or service designed to assist users in making informed decisions about fast food choices. This tool leverages data and algorithms to personalize recommendations based on user preferences, dietary restrictions, location, and other relevant factors. It aims to streamline the often overwhelming process of selecting a meal from a vast array of options, promoting a more efficient and potentially healthier approach to fast food consumption.
Core Functionality of a “Fast Food Chooser”
The fundamental functions of a “Fast Food Chooser” revolve around data processing and user interaction. These functionalities work in tandem to provide a personalized and effective decision-making experience.
- Restaurant Database and Menu Integration: A comprehensive database of fast-food restaurants is essential. This includes detailed menu information, nutritional facts (calories, fat, protein, carbohydrates, sodium), pricing, and often, allergen information. This data is usually obtained through APIs, web scraping, or direct partnerships with restaurants. The database needs constant updating to reflect menu changes and new restaurant openings. For example, a system might update its database daily, pulling data from public sources to ensure accuracy.
- User Profiling and Preference Management: The application allows users to create profiles and specify their preferences. This can include dietary restrictions (vegetarian, vegan, gluten-free, allergies), preferred cuisines, budget limitations, and taste preferences (e.g., spicy, sweet, savory). The system can also learn from a user’s past choices to refine future recommendations. For example, a user who consistently chooses low-sodium options will likely receive recommendations favoring those types of meals.
- Location-Based Services: Utilizing the user’s location (via GPS or IP address), the “Fast Food Chooser” identifies nearby restaurants. This is crucial for practicality, as it limits the choices to accessible options. The service can also incorporate distance and travel time estimates. This functionality frequently uses mapping APIs (e.g., Google Maps API) to display restaurant locations and provide directions.
- Recommendation Engine: The core of the application is the recommendation engine. This algorithm processes the user’s profile, menu data, and location information to generate personalized meal suggestions. The engine might use various techniques, including collaborative filtering (recommending items similar to what other users with similar preferences have chosen), content-based filtering (recommending items based on the user’s stated preferences and the item’s characteristics), and hybrid approaches.
- Filtering and Sorting Options: Users need tools to refine their search. These options typically include filtering by dietary restrictions, price range, cuisine type, and restaurant rating. Sorting options, such as by distance, price, or popularity, also enhance usability.
- Review and Rating Integration: Integrating reviews and ratings from other users is important for decision-making. This allows users to gauge the popularity and quality of restaurants and specific menu items. This data is often aggregated from third-party sources, such as Yelp or Google Reviews.
User Experience in a “Fast Food Chooser” Interaction
The user experience (UX) is critical to the success of a “Fast Food Chooser.” A well-designed interface ensures ease of use and promotes user engagement. A typical interaction would follow a sequence of steps.
Obtain recommendations related to daves dog food that can assist you today.
- Initial Setup and Profile Creation: The user opens the application or service. If it’s their first time, they create a profile. This involves providing basic information and specifying preferences. For example, the user might select “vegetarian” and set a budget limit of $10.
- Location Detection: The application detects the user’s location, either automatically or by prompting the user to enter an address or enable location services.
- Filtering and Customization: The user can apply filters based on their preferences. They might filter by cuisine (e.g., “burgers,” “Mexican”), dietary restrictions (e.g., “gluten-free”), or price range.
- Recommendation Display: The application presents a list of recommended restaurants and menu items, often sorted by relevance or distance. Each recommendation typically includes:
- Restaurant Name and Address
- Menu Item Name and Description
- Price
- Nutritional Information (calories, etc.)
- User Ratings and Reviews
- A photo of the menu item.
- Detailed Information and Selection: The user can tap on a recommendation to view more detailed information about the menu item. This might include ingredient lists, allergen information, and more in-depth reviews. The user then makes a selection.
- Ordering (Optional): Some “Fast Food Chooser” applications integrate directly with online ordering systems. This allows the user to place an order directly through the app.
- Feedback and Review: After the meal, the user might be prompted to provide feedback on their experience, which can improve the accuracy of future recommendations.
For example, a user might open a “Fast Food Chooser” app, indicate they are in a specific location, and specify a preference for “healthy” and “vegetarian” options. The app then presents a list of nearby restaurants offering vegetarian options that meet their criteria, displaying nutritional information and user reviews for each item. This streamlined process helps the user make a quick and informed decision, saving time and effort.
Target Audience & User Personas
Understanding the intended audience is critical for the success of the “Fast Food Chooser.” This involves defining specific user personas and identifying the broader demographics and psychographics that represent the primary target market. Furthermore, adaptability to various dietary needs and preferences is essential to broaden the application and appeal of the tool.
User Personas
Developing detailed user personas allows for a more targeted approach to design and development. These personas represent distinct user groups, each with unique needs and expectations.
- Persona 1: The Busy Professional (Sarah, 32) Sarah is a marketing manager who works long hours. She often has limited time for lunch and dinner, making fast food a convenient option. She prioritizes speed and efficiency, but also seeks healthier choices when available. Sarah uses her smartphone extensively for ordering and navigation. Her main concern is time-saving, with a secondary focus on health-conscious options.
She appreciates features like quick ordering, calorie information, and the ability to customize her meals. She is likely to be influenced by online reviews and recommendations.
- Persona 2: The Budget-Conscious Student (Mark, 20) Mark is a college student with a limited budget. He is looking for affordable meal options and is less concerned about nutritional value than Sarah. He often eats with friends and is interested in deals and discounts. Mark is active on social media and relies on apps for information and entertainment. His primary need is affordability, followed by convenience.
He values features like coupon integration, loyalty programs, and the ability to compare prices across different fast-food restaurants.
- Persona 3: The Health-Conscious Family (David & Emily, 45 & 43) David and Emily are parents with two children. They are health-conscious and want to make informed choices for their family. They are looking for options that are both nutritious and appealing to their children. They are willing to spend slightly more for healthier ingredients. They utilize online resources for nutritional information and are influenced by dietary guidelines and recommendations.
Their needs are centered around nutritional transparency, kid-friendly options, and ease of meal planning. They appreciate features like allergen filters, nutritional breakdowns, and the ability to compare healthy alternatives.
Demographics and Psychographics of the Primary Target Audience
Defining the target audience involves considering both demographic and psychographic factors to understand the characteristics of potential users.
- Demographics: The primary target audience for the “Fast Food Chooser” is likely to include individuals aged 18-55, with a slightly higher concentration in urban and suburban areas. Income levels would vary, reflecting the wide range of fast-food consumers. Educational backgrounds could also vary, encompassing a broad spectrum from high school graduates to those with advanced degrees. Geographic location is also important, with a higher concentration in areas with a dense presence of fast-food restaurants.
- Psychographics: Psychographically, the target audience can be segmented into several groups. The “Convenience Seekers” prioritize speed and ease of use. The “Value Shoppers” focus on affordability and deals. The “Health-Conscious Consumers” are concerned about nutritional value and ingredient quality. The “Social Eaters” often dine with friends or family and value social experiences.
Understanding these psychographic profiles allows for tailored marketing and feature development. For example, the tool could incorporate features like a ‘healthy options’ filter, a ‘deals and discounts’ section, or social sharing capabilities to cater to different psychographic segments.
Adaptability to Diverse Dietary Needs and Preferences
The “Fast Food Chooser” must accommodate a wide range of dietary requirements and preferences to maximize its appeal.
- Dietary Restrictions: The tool can incorporate filters for common dietary restrictions such as vegetarian, vegan, gluten-free, and allergies. Users should be able to easily identify menu items that meet their specific needs. This involves integrating comprehensive nutritional information and allergen data for each item.
- Dietary Preferences: The application can be adapted to cater to specific dietary preferences, such as low-carb, keto, or paleo diets. This could involve suggesting alternative meal combinations or highlighting menu items that align with these preferences. Users can be given the option to select their dietary preferences and receive personalized recommendations.
- Customization and Flexibility: The ability to customize meals is crucial. The “Fast Food Chooser” should allow users to modify existing menu items, such as requesting no cheese or extra vegetables. It should also provide detailed nutritional breakdowns for customized meals. The tool could provide suggestions for meal modifications based on user dietary needs and preferences.
Feature Set & Functionality
The “Fast Food Chooser” needs a robust set of features to provide a valuable and user-friendly experience. These features are essential for helping users make informed decisions about their fast-food choices, considering factors like location, dietary needs, and nutritional information. The following list Artikels the core functionalities required for such an application.
Essential Features
The following features are critical to the success of a “Fast Food Chooser,” ensuring it provides a comprehensive and helpful service.
- Restaurant Database: A comprehensive and regularly updated database of fast-food restaurants, including their menus, hours of operation, and contact information. This is the foundation upon which the application is built.
- Menu Browsing: The ability to browse menus for individual restaurants, with clear categorization of food items (e.g., burgers, fries, salads). This should include high-quality images of the food items to enhance the user experience.
- Search Functionality: Robust search capabilities allowing users to search for specific menu items, restaurants, or ingredients. This could include advanced search filters (e.g., price range, dietary restrictions).
- Nutritional Information: Detailed nutritional information for each menu item, including calories, fat, carbohydrates, protein, and sodium content. This should be sourced from reliable databases like the USDA FoodData Central.
- User Accounts: User accounts to save preferences, dietary restrictions, favorite restaurants, and order history. This personalization enhances user engagement and satisfaction.
- Restaurant Reviews and Ratings: Integration of user reviews and ratings for restaurants, providing valuable social proof and helping users make informed decisions. This could be integrated with services like Yelp or Google Reviews.
- Location-Based Services: Integration with location services to identify nearby restaurants and provide directions. This is crucial for the convenience of the user.
- Order Placement Integration (Optional): The ability to place orders directly through the app, potentially integrating with existing fast-food ordering platforms or allowing for direct ordering.
- Customizable Filters: Filters based on dietary restrictions, price, and cuisine type, to streamline the user experience.
- Accessibility Features: Ensuring the application is accessible to users with disabilities, including screen reader compatibility and adjustable font sizes.
Location-Based Services Integration
Location-based services are crucial for the “Fast Food Chooser” to provide a relevant and convenient user experience. This integration allows the app to identify nearby restaurants and tailor recommendations based on the user’s current location.The application would leverage the device’s GPS or Wi-Fi triangulation to determine the user’s location. This information is then used to:
- Identify Nearby Restaurants: The app displays a list of fast-food restaurants within a specified radius of the user’s location. The distance from the user’s location to each restaurant is displayed.
- Provide Directions: Integration with mapping services (e.g., Google Maps, Apple Maps) allows users to get directions to a selected restaurant. This enhances convenience, especially for users who are unfamiliar with the area.
- Offer Location-Specific Promotions: The app could display location-specific promotions or deals offered by nearby restaurants. This adds value for the user and incentivizes them to use the app.
- Filter Results by Distance: Users can filter the search results by distance, prioritizing restaurants closest to their location.
For example, imagine a user is in downtown Chicago. The “Fast Food Chooser” would identify all nearby restaurants (McDonald’s, Burger King, Chipotle, etc.). The app would then display a map showing the location of each restaurant, along with their distance from the user’s current location. Clicking on a restaurant would provide detailed information, including directions, menu, and nutritional information.
Handling Nutritional Information and Dietary Restrictions
Providing accurate and accessible nutritional information, along with the ability to filter for dietary restrictions, is a critical aspect of the “Fast Food Chooser.” This functionality empowers users to make informed and healthy choices.The application would incorporate the following features to address nutritional information and dietary restrictions:
- Comprehensive Nutritional Data: The app would provide detailed nutritional information for each menu item, including calories, fat (saturated, trans), carbohydrates (sugars, fiber), protein, and sodium. This data would be sourced from reputable databases such as the USDA FoodData Central or direct feeds from restaurant chains, ensuring accuracy and consistency.
- Clear and Concise Presentation: Nutritional information would be presented in a clear and easy-to-understand format. This could include tables, charts, or visual representations of the nutritional content.
- Dietary Restriction Filters: Users would be able to specify dietary restrictions, such as:
- Vegetarian
- Vegan
- Gluten-free
- Dairy-free
- Nut allergies
- Low-sodium
- Low-carb
The app would then filter the search results to only show menu items that meet the specified dietary requirements.
- Ingredient Information: The app could provide a list of ingredients for each menu item, allowing users to make informed decisions based on their sensitivities or preferences.
- Customizable Meal Planning: Users could potentially create custom meal plans, based on their dietary needs and preferences, tracking their daily nutritional intake.
- Alerts and Warnings: The app could provide alerts or warnings if a menu item contains ingredients that a user has specified as restricted (e.g., “Contains nuts”).
For instance, a user with a gluten intolerance could set a “gluten-free” filter. The app would then only display menu items that are confirmed to be gluten-free, such as certain salads or grilled chicken sandwiches (without the bun). The nutritional information for these items would be clearly displayed, allowing the user to assess the calorie and macronutrient content.
Data Sources & Information Gathering: Fast Food Chooser
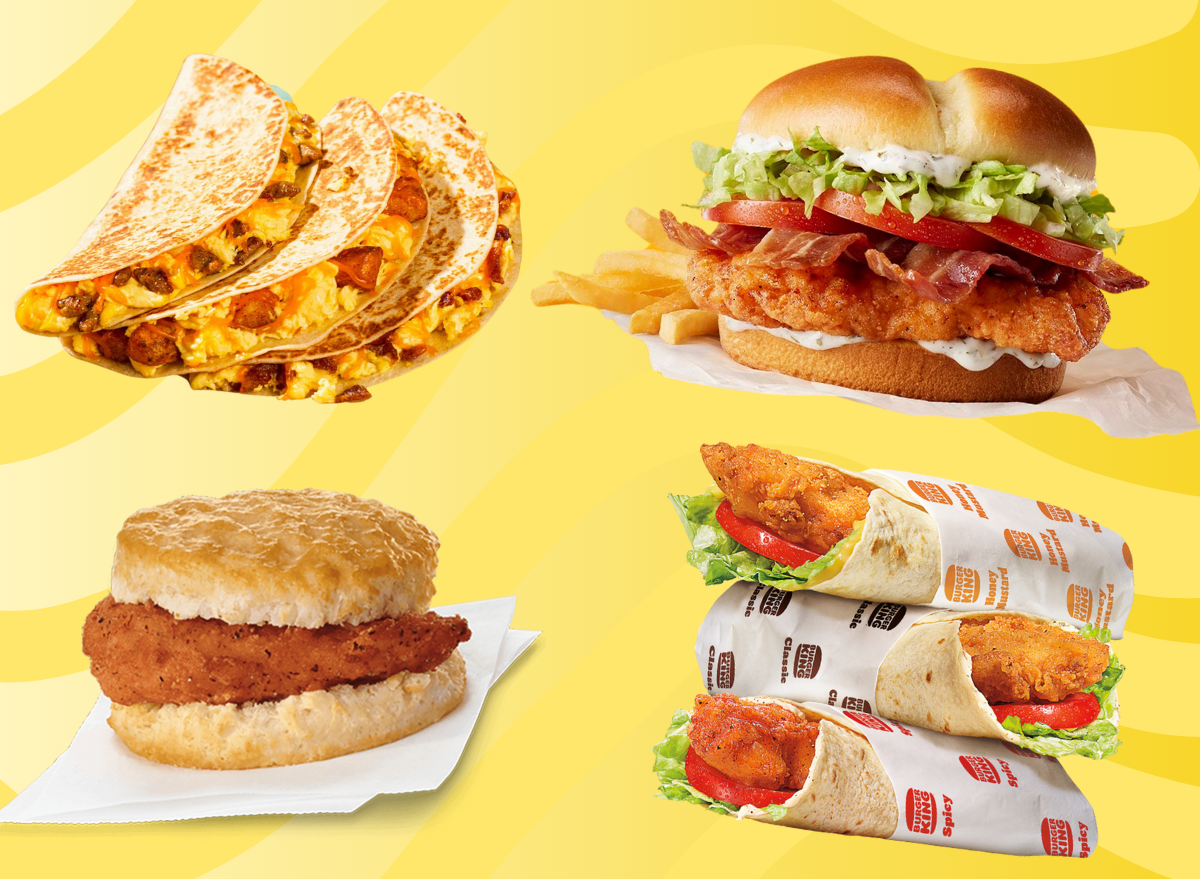
The “Fast Food Chooser” application’s success hinges on its ability to access and process accurate, up-to-date information. This necessitates a robust system for data acquisition from various sources, coupled with rigorous methods to ensure data integrity and currency. The following sections detail the crucial data sources, accuracy measures, and challenges associated with building a reliable fast-food information platform.
Primary Data Sources
The application relies on several primary data sources to provide comprehensive fast-food information. These sources contribute to the application’s ability to offer a wide range of features, from menu comparisons to nutritional analysis.
- Restaurant Websites and APIs: This is a crucial source. Many fast-food chains provide menus, nutritional information, and pricing on their websites. Some also offer Application Programming Interfaces (APIs) that allow for direct data access. Using APIs is preferable because it offers a structured way to retrieve data, improving the efficiency of the application.
- Third-Party Data Providers: Companies specializing in restaurant data aggregation are another vital source. These providers collect and maintain data from various sources, including restaurant websites, menus, and user-submitted information. They often offer standardized data formats, making it easier to integrate data into the application. Examples include platforms like MenuLog, or similar services that consolidate restaurant listings.
- User-Generated Content (UGC): Information provided by users is essential for real-time updates, such as restaurant hours, special offers, and customer reviews. The application can incorporate user-submitted data through feedback forms, review sections, and crowdsourcing mechanisms. This UGC data provides the application with up-to-date information on restaurant hours, deals, and user experiences.
- Point of Sale (POS) Systems (Indirectly): While direct access to POS systems is unlikely, the application can leverage publicly available information regarding menu changes, and promotional offers often announced through press releases or marketing materials. This indirectly offers insights into real-time updates.
Data Accuracy and Up-to-Date Information
Maintaining data accuracy and currency is paramount for the “Fast Food Chooser” application. Several strategies are employed to achieve this goal, ranging from automated checks to human verification.
- Automated Data Validation: The application employs automated validation routines to check data consistency and integrity. This includes checking for data type errors (e.g., ensuring that nutritional values are numeric) and range violations (e.g., ensuring that calorie counts fall within a reasonable range). Automated validation reduces the chance of inaccurate information being displayed to users.
- Data Scraping and Parsing Techniques: Data scraping techniques, used to extract information from restaurant websites, are continuously refined. These techniques must be robust to handle changes in website structure. The application uses parsing libraries to interpret and structure the scraped data correctly.
- Real-Time Updates and Synchronization: To keep the application’s information current, real-time updates and synchronization mechanisms are essential. This involves regularly polling data sources, comparing data with existing information, and updating the database accordingly. Real-time updates can be facilitated by APIs or webhooks that push changes as they occur.
- User Feedback and Verification: Incorporating user feedback and verification mechanisms is a key part of data accuracy. Users can report inaccuracies, suggest corrections, and provide reviews that help validate information. The application can use algorithms to identify and prioritize user-submitted data for review, increasing the accuracy of information displayed.
- Version Control and Audit Trails: Implementing version control and audit trails is a standard practice in database management. The application tracks data changes over time, allowing for the identification of errors and the restoration of previous data versions. Audit trails are essential for investigating data inconsistencies and ensuring data quality.
- Example: Consider a scenario where a fast-food restaurant introduces a new menu item. The application would first detect this change by scraping the restaurant’s website or receiving an update through an API. Then, the data validation processes would confirm the new item’s details. Finally, user reviews would help verify the accuracy of the information.
Challenges in Data Acquisition and Maintenance
Acquiring and maintaining data from fast-food restaurants presents several challenges. These challenges require careful planning and proactive solutions to ensure the application’s long-term success.
- Website Structure Changes: Fast-food restaurants frequently update their websites, altering the structure of menus and other information. This necessitates constant adjustments to data scraping and parsing techniques. These changes can break data extraction processes, requiring the development team to regularly adapt the application.
- Data Standardization and Format Differences: Different fast-food chains use various data formats and presentation styles, making it difficult to standardize data across all restaurants. The application must accommodate these differences through data mapping and transformation processes.
- API Limitations and Access Restrictions: Some fast-food chains may not offer public APIs, limiting access to their data. Even when APIs are available, they may have rate limits or other restrictions that affect the application’s ability to collect and update data efficiently.
- Data Accuracy from Third-Party Providers: While third-party data providers offer a convenient source of information, the accuracy of their data is not always guaranteed. The application needs to validate data from these providers and implement measures to correct errors.
- Data Volume and Scalability: The fast-food industry generates a large volume of data, including menus, nutritional information, and pricing. The application’s database and infrastructure must be scalable to handle this volume and maintain acceptable performance.
- Legal and Ethical Considerations: The application must comply with legal and ethical requirements regarding data privacy and usage. It must also respect the intellectual property rights of fast-food restaurants and other data providers.
- Example: If a restaurant changes its menu, the application must quickly adapt its data extraction process. The application should monitor the website for changes and use parsing libraries to correctly structure the updated data. This will ensure the application continues to provide accurate and up-to-date information to its users.
Comparison & Recommendation Algorithms
The core of the “Fast Food Chooser” lies in its ability to intelligently compare fast-food options and provide personalized recommendations. This functionality is powered by sophisticated algorithms that analyze vast amounts of data and user preferences. The algorithms employ a combination of techniques to ensure accurate and relevant suggestions, considering factors ranging from nutritional information to user-specific taste profiles.
Methods for Comparing Fast-Food Options
Comparing fast-food options requires a multifaceted approach, encompassing nutritional content, price, customer ratings, and proximity. The algorithm employs several methods to achieve this comparison effectively.
- Nutritional Content Analysis: The system utilizes a database of nutritional information for each menu item, typically sourced from the fast-food chains themselves or third-party databases like the USDA National Nutrient Database. The comparison involves analyzing macronutrients (calories, protein, carbohydrates, and fats), micronutrients (vitamins and minerals), and potentially undesirable components like sodium and added sugars. For example, the algorithm might compare two burgers based on their saturated fat content, providing a score or rating reflecting the healthiness of each option.
- Price Comparison: The algorithm tracks the prices of menu items from different fast-food chains. Price data can be obtained through various means, including scraping restaurant websites, utilizing publicly available pricing APIs (if available), and user-submitted price updates. The algorithm calculates the cost per serving, or the cost per nutritional value, to allow for a more informed comparison.
- Customer Rating Aggregation: The system aggregates customer ratings and reviews from various sources, such as online review platforms (e.g., Yelp, Google Reviews), and social media. These ratings are often weighted based on factors like the source’s reliability and the recency of the review. The algorithm calculates an average rating for each menu item, providing a measure of customer satisfaction.
- Proximity Assessment: The algorithm uses the user’s location (obtained through GPS or manual input) to identify nearby fast-food restaurants. It then calculates the distance to each restaurant, providing a factor for comparison based on convenience. This feature is particularly important for users who prioritize ease of access.
- Feature-Based Comparison: The algorithm can compare items based on specific features or characteristics. This allows for more granular comparisons, such as comparing the spiciness level of different chicken sandwiches, or the availability of vegetarian options. The data for these features is gathered from menu descriptions, user reviews, and potentially through the use of image recognition to analyze food items.
Algorithm for Personalized Recommendations
The recommendation engine uses a collaborative filtering and content-based filtering approach to provide personalized suggestions. This hybrid approach combines the strengths of both methods to deliver accurate and relevant recommendations.
- Collaborative Filtering: This technique identifies users with similar preferences (e.g., users who frequently order similar menu items or have similar rating patterns). When a new user enters the system, their preferences are compared to those of other users. Based on the preferences of similar users, the algorithm recommends items that the new user has not yet tried but which have been positively rated by their peers.
For instance, if two users consistently rate similar items highly, the algorithm is more likely to recommend an item that one user has tried and the other has not.
- Content-Based Filtering: This method recommends items based on the user’s past behavior and the characteristics of the items themselves. The algorithm analyzes the user’s order history, ratings, and preferences to build a profile. It then recommends items that share similar characteristics with the items the user has previously enjoyed. For example, if a user frequently orders spicy chicken sandwiches, the algorithm is more likely to recommend other spicy chicken sandwiches or items with similar flavor profiles.
- Preference Weighting: The algorithm allows for the weighting of different factors to personalize recommendations further. Users can specify the importance of factors such as healthiness, price, or speed of service. For example, a user prioritizing health may assign a higher weight to the nutritional content of menu items, causing the algorithm to prioritize lower-calorie, lower-fat options.
- A/B Testing and Machine Learning: The recommendation engine can be continuously improved through A/B testing, where different recommendation algorithms are tested on different user groups. Machine learning techniques are used to refine the algorithm over time, learning from user behavior and feedback to improve the accuracy and relevance of the recommendations.
Handling Conflicting User Preferences
The recommendation engine is designed to handle conflicting user preferences gracefully. This involves balancing different priorities and providing options that cater to a diverse range of needs.
- Compromise Recommendations: When users have conflicting preferences (e.g., one user values healthiness while the other prioritizes taste), the algorithm might suggest compromise options. For instance, it could recommend a smaller portion of a less healthy item or a healthier alternative that still satisfies the user’s craving.
- Preference Override: The system provides mechanisms for users to override the algorithm’s recommendations if they are not satisfied. Users can manually search for specific items, filter results based on certain criteria, or provide feedback on the recommendations they receive.
- User Profiling and Segmentation: The algorithm segments users into different profiles based on their preferences and behavior. This allows for the creation of more targeted recommendations. For example, a user who frequently orders unhealthy items might be placed in a “indulgence” profile, while a user who consistently chooses healthy options might be placed in a “health-conscious” profile. The algorithm tailors recommendations based on the profile the user is assigned to.
- Transparency and Explainability: The system provides transparency into why certain recommendations are made. This allows users to understand the reasoning behind the suggestions and adjust their preferences accordingly. The algorithm might highlight the specific factors that influenced a recommendation (e.g., “Based on your past orders of spicy chicken sandwiches, we recommend…”).
User Interface & Design
The user interface (UI) of the Fast Food Chooser is paramount for its success. A well-designed UI ensures that users can easily navigate the application, understand the presented information, and make informed decisions efficiently. The visual appeal of the interface also plays a crucial role in user engagement and satisfaction. This section Artikels the design principles and implementation strategies for a user-friendly and visually engaging fast-food selection tool.
Interface Elements Organization
The organization of interface elements is designed to guide the user through the decision-making process in a logical and intuitive manner. The core principle is to minimize cognitive load, allowing users to focus on their preferences and the available options.The main screen will feature a prominent search bar at the top, enabling users to quickly search for specific restaurants or menu items.
Below the search bar, a series of filters will be displayed. These filters, categorized for easy access, include: dietary restrictions (vegetarian, vegan, gluten-free), cuisine type (Mexican, Italian, American), price range, and distance from the user’s current location. A map view will visualize nearby fast-food restaurants, dynamically updating as filters are applied. Each restaurant on the map will be represented by a marker.
Clicking a marker reveals a brief summary of the restaurant, and tapping this summary will lead to the restaurant’s detailed page. The detailed page provides comprehensive information including menu items, nutritional information, user reviews, and restaurant ratings.
Responsive Design for Multiple Devices
Adaptability across various screen sizes and devices is a critical consideration. The application should maintain its functionality and visual appeal regardless of whether it is accessed on a smartphone, tablet, or desktop computer. This is achieved through responsive design principles.The UI is structured using HTML tables with responsive columns to accommodate different screen sizes. The use of CSS media queries allows for dynamic adjustments to the layout and content presentation.
Small Screens (Phones) | Medium Screens (Tablets) | Large Screens (Desktops) | Example |
---|---|---|---|
Filters displayed as a vertical list. | Filters displayed as a horizontal row, accessible through a scrolling interface. | Filters displayed as a sidebar or top navigation bar. | Imagine a mobile phone displaying the filter options as a list, allowing users to easily scroll through them on the smaller screen. |
Restaurant listings stacked vertically. | Restaurant listings displayed in a two-column grid. | Restaurant listings displayed in a three or four-column grid. | On a tablet, restaurant listings would appear in a two-column format, improving readability and making better use of screen space. |
Map view optimized for touch interaction. | Map view with larger touch targets. | Map view with standard mouse interaction. | A desktop user would be able to easily interact with the map using a mouse, while a tablet user would be able to touch the map with their fingers. |
Menu items presented with minimal detail, focusing on key information. | Menu items displayed with moderate detail, including images and brief descriptions. | Menu items presented with detailed information, high-resolution images, and nutritional information. | A desktop user would be able to see the full menu item descriptions, nutritional information, and large images. |
For example, the filter options might be displayed as a vertical list on smaller screens (smartphones) to optimize space, while on larger screens (desktops), they could be arranged horizontally or in a sidebar. Similarly, restaurant listings could adapt from a single-column layout on phones to a multi-column grid on tablets and desktops. The map view would also be responsive, with touch-optimized controls for mobile devices and standard mouse interaction for desktop users.
The visual elements, such as fonts and icons, will scale appropriately to maintain readability and clarity across all devices.
Content Creation and Presentation
The “Fast Food Chooser” is designed to be more than just a menu browser. It aims to empower users with the information they need to make informed and healthy choices. This section details how the tool presents its content and how users can effectively leverage its features.
Navigating the Tool
The “Fast Food Chooser” provides a user-friendly interface for navigating the available options. Understanding the tool’s structure ensures users can easily access the information they need.
- Search Functionality: Users can initiate their search by typing s (e.g., “burger,” “salad,” “vegetarian”) into the search bar. The tool then filters the database to display relevant items from various fast-food restaurants.
- Restaurant Selection: A dedicated section allows users to select specific restaurants, filtering the displayed menu items. This feature is particularly useful for those with specific restaurant preferences or dietary restrictions.
- Filtering Options: The tool incorporates filtering options based on dietary needs (e.g., vegan, gluten-free, low-carb) and nutritional parameters (e.g., calories, fat, protein). Users can combine these filters to narrow down their choices and find meals that align with their goals.
- Detailed Item Pages: Each menu item has its own page that displays comprehensive information. This includes the item’s name, description, price, and nutritional information, along with any relevant allergen information.
Providing Additional Information
Beyond basic menu listings, the “Fast Food Chooser” enhances its value by providing additional context and data. This comprehensive approach empowers users to make well-informed choices.
- Nutritional Breakdown: Each item page includes a detailed nutritional breakdown. This includes the number of calories, fat (saturated, trans, and unsaturated), carbohydrates (sugars and fiber), protein, and sodium. This data is sourced from the restaurants themselves or from reliable nutritional databases, such as the USDA FoodData Central.
- Ingredient Lists: The tool often includes ingredient lists for each item, allowing users to identify potential allergens or ingredients they may want to avoid. This transparency is particularly helpful for individuals with food sensitivities.
- Comparison Tools: The tool allows users to compare multiple menu items side-by-side. This comparison highlights the differences in nutritional content, enabling users to make informed decisions based on their dietary needs and preferences.
- Serving Size Guidance: Information about serving sizes is displayed clearly, helping users understand the actual portion size and associated nutritional values. This feature helps avoid overconsumption.
Making Informed Choices
The “Fast Food Chooser” is designed to assist users in making choices that align with their health goals. This involves providing information about the health aspects of various menu items.
- Health Scores: The tool may assign health scores to menu items based on nutritional profiles. These scores consider factors such as calorie count, fat content, sugar content, sodium levels, and fiber content. A higher score generally indicates a healthier choice.
- Dietary Recommendations: The tool may provide general dietary recommendations based on user preferences or profiles. For example, it might suggest lower-calorie options, meals with high fiber content, or items with reduced sodium.
- Health Alerts: The tool may issue alerts if a menu item contains excessive levels of certain nutrients, such as sodium or saturated fat. These alerts help users identify potentially unhealthy options.
- Examples:
- Scenario 1: A user searching for a “burger” might see a comparison between a standard cheeseburger and a grilled chicken sandwich. The tool would highlight the lower calorie and fat content of the grilled chicken sandwich, along with the higher protein content, allowing the user to make a more informed decision.
- Scenario 2: A user with a sodium restriction could use the filter function to identify low-sodium menu items. The tool would highlight options like salads with light dressing or grilled chicken without added salt, and would also provide the exact sodium content.
Monetization Strategies (if applicable)
The development of a “Fast Food Chooser” presents several opportunities for monetization, transforming the app from a helpful tool into a sustainable business. These strategies must be carefully considered to balance revenue generation with a positive user experience. The primary goal is to create value for both the users and the business.
Advertising Integration
Advertising is a common monetization strategy, and its success hinges on unobtrusiveness and relevance. The goal is to deliver advertisements that complement the user experience, not detract from it.
- Contextual Advertising: This involves displaying ads that are directly related to the user’s search criteria. For example, if a user is looking for “burgers near me,” the app could display ads for burger joints in the vicinity. This is more effective than generic ads. According to a study by HubSpot, users are 2.9 times more likely to click on ads that are relevant to their interests.
- Sponsored Listings: Restaurants can pay to have their listings appear higher in the search results or be featured in a “featured restaurants” section. This provides restaurants with increased visibility and the app with a revenue stream. The app should clearly label sponsored listings to maintain transparency.
- Non-Intrusive Display Ads: Banner ads can be integrated into the app’s interface, but their placement is critical. They should be located in areas where they don’t interfere with the core functionality. For example, a banner ad at the bottom of the search results page. A study by Google showed that non-intrusive banner ads have a higher click-through rate compared to more aggressive ad formats.
- Video Ads (with user control): Short, skippable video ads can be shown between searches or when the user is viewing restaurant details. Allowing users to skip the ads after a few seconds enhances user experience.
Premium Subscription Model
A premium subscription model can offer enhanced features that appeal to users who want a more comprehensive experience. These features should provide significant value to justify the subscription cost.
- Advanced Filtering Options: Subscribers could gain access to more granular filtering options, such as specific dietary requirements (e.g., low-sodium, organic ingredients) or advanced search criteria (e.g., “best fries” or “restaurants with drive-thru”).
- Exclusive Deals and Discounts: Partnering with restaurants to offer exclusive deals and discounts to subscribers can be a significant incentive. This could include percentage-off discounts, free items, or early access to promotions.
- Offline Functionality: Allowing subscribers to access restaurant information, search results, and saved favorites offline can be a valuable feature, particularly for users who travel or have limited internet access.
- Ad-Free Experience: A key benefit for subscribers could be an ad-free experience, removing all advertisements from the app. This enhances usability and can significantly improve user satisfaction.
- Personalized Recommendations: Premium subscribers could receive more personalized recommendations based on their past searches, ratings, and dietary preferences. This could involve using more sophisticated recommendation algorithms.
Other Potential Revenue Streams
Additional strategies can diversify revenue sources and strengthen the business model.
- Affiliate Marketing: Partnering with food delivery services and earning a commission on orders placed through the app.
- Data Analytics: Offering anonymized and aggregated data insights to restaurants, such as popular menu items, peak hours, and customer preferences. This can provide valuable market research data.
- In-App Purchases: Offering virtual items or add-ons, such as aesthetic customizations, may generate revenue.
Marketing and Promotion
The successful launch and sustained growth of the “Fast Food Chooser” hinges on a robust marketing and promotion strategy. This plan must effectively reach the target audience, highlight the application’s unique value proposition, and drive user acquisition and engagement. The following sections detail key components of this strategy, leveraging diverse channels and tactics.
Marketing Plan for Target Audience Engagement
A multi-faceted marketing plan is crucial for reaching the target audience effectively. This plan encompasses various strategies, each tailored to maximize reach and impact.
- Social Media Marketing: Utilize platforms like Instagram, TikTok, and Facebook to showcase the application’s features through engaging content. This includes short video demonstrations, user testimonials, and visually appealing infographics. Run targeted ad campaigns based on demographic data, location, and interest profiles to reach the desired user base. A/B test different ad creatives to optimize for click-through rates and conversions.
- Search Engine Optimization (): Optimize the application’s website and app store listings with relevant s to improve organic search rankings. Conduct research to identify terms that users are likely to search for when looking for fast food recommendations or comparisons. Regularly update content to maintain high search engine visibility.
- Content Marketing: Create valuable content, such as blog posts, articles, and guides, related to fast food, nutrition, and restaurant reviews. Share this content across social media and other platforms to attract and engage potential users. Partner with food bloggers and influencers to create sponsored content and reviews.
- Public Relations: Reach out to food critics, journalists, and bloggers to secure media coverage and generate positive reviews. Prepare press releases announcing the application’s launch and updates. Participate in industry events and conferences to build brand awareness and network with potential partners.
- Email Marketing: Build an email list through website sign-ups and in-app prompts. Send newsletters with application updates, exclusive deals, and personalized recommendations based on user preferences. Segment the email list to target specific user groups with tailored content.
- App Store Optimization (ASO): Optimize the application’s app store listing with relevant s, compelling descriptions, and high-quality screenshots. Encourage users to leave positive reviews and ratings to improve app store visibility.
Effective Social Media Marketing Campaigns
Social media platforms offer powerful tools for reaching the target audience. Successful campaigns on these platforms require creativity, consistency, and data-driven optimization.
- Instagram Campaign Example: Create a series of visually appealing short videos showcasing different fast-food items and how the “Fast Food Chooser” can help users make informed decisions. Partner with food photographers to create high-quality images. Run contests and giveaways to encourage user engagement. Use relevant hashtags like #fastfood, #foodie, #restaurantreview, and #foodcomparison. Consider a campaign based on a specific diet type, like “Keto Fast Food Finds” to attract a niche audience.
This could include posts highlighting low-carb options and recommendations.
- TikTok Campaign Example: Leverage TikTok’s short-form video format to create engaging content. Develop challenges like “Fast Food Challenge” where users try to find the best value meal or healthiest option using the app. Partner with TikTok influencers who focus on food or lifestyle content. Create trending sounds and utilize them in the app’s videos to increase visibility. This strategy aligns with TikTok’s algorithm and can significantly boost reach.
- Facebook Campaign Example: Run targeted Facebook ad campaigns to reach specific demographics and interests. Create a Facebook group for users to share their experiences and recommendations. Share blog posts and articles related to fast food and nutrition. Host live Q&A sessions with nutritionists or food experts. A campaign might focus on comparing the nutritional value of similar meals at different restaurants, providing data-driven insights to help users make healthier choices.
Leveraging Partnerships with Fast-Food Restaurants for Promotion
Collaborations with fast-food restaurants can significantly boost the application’s visibility and user base. These partnerships can provide mutual benefits, increasing reach for both parties.
- Co-branded Promotions: Partner with restaurants to offer exclusive deals and discounts to “Fast Food Chooser” users. This could involve integrating the app with the restaurant’s ordering system or providing coupons for specific menu items. A partnership with a popular burger chain could offer a “Fast Food Chooser” recommended combo meal at a discounted price.
- In-App Integration: Integrate the application with restaurant loyalty programs or online ordering systems. This would allow users to directly order from the app and earn rewards points. The app could include a button to seamlessly navigate to the restaurant’s online ordering platform.
- Content Collaboration: Collaborate with restaurants to create sponsored content, such as blog posts or videos, showcasing their menu items and highlighting the “Fast Food Chooser’s” features. This could involve restaurant-sponsored recipe ideas that users can create, and they can use the app to search for ingredients.
- Data Sharing (with consent): Offer restaurants aggregated and anonymized user data, such as popular menu items, dietary preferences, and customer ratings. This data can help restaurants improve their offerings and marketing strategies. Ensure compliance with all privacy regulations.
- Cross-promotion: Promote the application on restaurant websites, social media channels, and in-store materials. Display QR codes or links to download the app in restaurants. Display the app’s logo on restaurant menus or promotional materials.
Technical Implementation Considerations
Building a “Fast Food Chooser” application necessitates careful consideration of various technical aspects to ensure a user-friendly, scalable, and efficient experience. The core of the application’s functionality relies on a robust technical foundation capable of handling data ingestion, processing complex algorithms, and presenting information effectively. This section delves into the crucial technical considerations for realizing this vision.
Platform and Technologies
Selecting the appropriate platform and technologies is paramount for the application’s success. The choice impacts development time, cost, scalability, and maintainability. Several options exist, each with its own advantages and disadvantages.
- Platform Options: The application could be built as a native mobile app (iOS, Android), a web application (accessible through a browser), or a hybrid application (combining aspects of both).
- Native Mobile Apps: Offer optimal performance and access to device features but require separate codebases for iOS and Android. Languages like Swift (iOS) and Kotlin (Android) are typically used.
- Web Applications: Provide cross-platform compatibility and are easily accessible but might have limitations regarding device features. Technologies like HTML, CSS, and JavaScript are used, often with frameworks like React, Angular, or Vue.js.
- Hybrid Apps: Use web technologies within a native wrapper, offering a balance between cross-platform compatibility and device feature access. Frameworks like React Native or Flutter are popular choices.
- Backend Technologies: The backend is responsible for data storage, processing, and serving APIs.
- Programming Languages: Python (with frameworks like Django or Flask), Node.js (with Express.js), Java (with Spring), and Ruby on Rails are common choices.
- Database: A database stores information about fast food restaurants, menus, nutritional information, and user preferences. Options include relational databases (PostgreSQL, MySQL) and NoSQL databases (MongoDB, Cassandra). The choice depends on the data structure and scalability requirements. For instance, MongoDB is suitable for storing unstructured data, while PostgreSQL offers robust support for complex queries.
- API Development: APIs (Application Programming Interfaces) allow the frontend to communicate with the backend. RESTful APIs are a common approach, using JSON for data exchange.
- Frontend Technologies: The frontend is the user interface.
- Programming Languages: HTML, CSS, and JavaScript are fundamental.
- Frameworks: Frameworks like React, Angular, or Vue.js streamline development, provide reusable components, and enhance user experience.
- State Management: Libraries such as Redux or Vuex manage application state, crucial for complex interactions.
Scalability and Performance
Scalability and performance are critical for handling a growing user base and ensuring a responsive user experience. The application must be designed to accommodate increasing traffic and data volumes without performance degradation.
- Database Optimization: Efficient database design and query optimization are crucial. Indexing frequently queried fields and using appropriate data types improve query performance. For example, using a geospatial index for restaurant locations allows for faster proximity searches.
- Caching: Implementing caching mechanisms reduces server load and improves response times.
- Caching Layers: Utilize caching layers like Redis or Memcached to store frequently accessed data, such as restaurant details or menu items. This significantly reduces the load on the database.
- Content Delivery Network (CDN): A CDN distributes static assets (images, CSS, JavaScript) across multiple servers geographically closer to users, reducing latency.
- Load Balancing: Distributing traffic across multiple servers ensures high availability and prevents overload. Load balancers distribute incoming requests across multiple backend servers, ensuring no single server is overwhelmed.
- Asynchronous Tasks: Offloading time-consuming tasks to background processes improves responsiveness. For example, generating personalized recommendations or sending email notifications can be handled asynchronously.
- Code Optimization: Writing efficient and optimized code is essential. Profiling tools identify performance bottlenecks, allowing developers to optimize code and algorithms. Techniques like code minification and bundling reduce file sizes and improve loading times.
- Monitoring and Alerting: Continuous monitoring of application performance is vital. Tools like Prometheus and Grafana provide real-time insights into server performance, error rates, and user behavior. Alerting systems notify developers of critical issues, allowing for rapid response.
The implementation of these technical considerations will result in a fast, reliable, and user-friendly “Fast Food Chooser” application capable of scaling to meet the demands of a large and active user base. The application must be designed to handle large amounts of data and provide accurate recommendations in real-time.
Illustrations and Visuals
Visual representations are crucial for a “Fast Food Chooser” to effectively communicate complex information quickly and accessibly. The design should prioritize clarity and ease of understanding, enabling users to make informed decisions efficiently. Effective use of visuals can significantly enhance user engagement and satisfaction.
Nutritional Information Representation
Visualizing nutritional data allows users to instantly grasp the health implications of their choices. Instead of relying solely on numerical data, the application can leverage graphical elements to convey the information more intuitively.
- Calorie Display: Calories can be represented using a progress bar. The bar fills up proportionally to the calorie content of the selected item. A full bar could indicate a high-calorie meal, while a partially filled bar suggests a lower calorie option. For example, a burger with 500 calories might fill the bar halfway if the bar’s maximum capacity is 1000 calories.
- Fat Content: Fat content can be illustrated with a pie chart or a donut chart. The size of the colored section representing fat content will be proportional to the grams of fat in the food item. The chart’s color can also be adjusted based on the type of fat: saturated fat could be a different color from unsaturated fat, allowing for easy differentiation.
- Sodium Levels: Sodium can be depicted using a gauge-like visual. The needle on the gauge points towards a high, medium, or low sodium level, allowing users to quickly assess the salt content. Color-coding can further enhance understanding; for instance, red could indicate high sodium, yellow medium, and green low.
- Sugar Content: Sugar content can be displayed with a series of sugar cubes or a similar visual representation. The number of sugar cubes would visually represent the amount of sugar in the item. For example, a soda with 40 grams of sugar could be represented by 10 sugar cubes, each representing 4 grams.
- Overall Health Score: An overall health score, derived from the nutritional data, can be shown using a star rating system or a simple numerical score. A higher score would indicate a healthier option. For instance, a meal could receive a score from 1 to 5 stars, with 5 stars representing the healthiest choice.
Dietary Restriction Icons and Symbols, Fast food chooser
Icons and symbols are essential for conveying dietary information quickly and clearly, particularly for users with specific dietary needs.
- Vegetarian: A green leaf or a stylized vegetable icon can signify vegetarian-friendly options. This is a universally recognized symbol, making it easily understood by users worldwide.
- Vegan: A vegan icon, often a stylized V with a circle or a plant-based symbol, can indicate vegan-friendly items. This clearly differentiates between vegetarian and vegan options.
- Gluten-Free: A crossed-out wheat symbol is the standard for gluten-free labeling. This icon immediately informs users about the absence of gluten.
- Nut-Free: A nut-free symbol, often a crossed-out nut or a simplified representation of a nut, is used to indicate the absence of nuts and potential cross-contamination.
- Dairy-Free: A crossed-out milk carton or a similar symbol indicates dairy-free options. This is essential for users with lactose intolerance or dairy allergies.
- Halal/Kosher: Specific symbols can be incorporated to indicate adherence to religious dietary laws. These symbols provide crucial information for users following Halal or Kosher guidelines.
Mobile User Interface Illustration Guide
A mobile user interface for a “Fast Food Chooser” should be intuitive and user-friendly. Here’s a descriptive guide for creating an illustration of the application’s interface.
- Screen Layout: The illustration should depict a smartphone screen, oriented vertically. The top of the screen should feature the app’s name and logo, for example, “Fast Food Finder” in a clean, readable font.
- Search Bar: At the top or below the logo, there should be a prominent search bar. This bar allows users to enter search terms like “burger,” “pizza,” or a specific restaurant name. The search bar should have a magnifying glass icon on the left and a clear “Search” prompt.
- Filter and Sort Options: Below the search bar, provide icons representing filters. These can include icons for dietary restrictions (vegetarian, vegan, gluten-free), price range (dollar signs), and health metrics (low calorie, low fat). There should also be a “Sort by” option, allowing users to sort results by relevance, price, or health score.
- Food Item Cards: The main area of the screen should display food items in a card format. Each card should contain the following:
- Image: A clear, high-quality image of the food item.
- Name: The name of the food item (e.g., “Big Mac”).
- Restaurant Name: The name of the restaurant offering the item (e.g., “McDonald’s”).
- Brief Nutritional Information: A concise summary of key nutritional information. This could include a calorie count (e.g., “500 Calories”), fat content (e.g., “25g Fat”), and a star rating for health score.
- Dietary Restriction Icons: If applicable, include the appropriate icons for dietary restrictions (vegetarian, vegan, gluten-free).
- Price: The price of the food item.
- Card Interaction: The card design should allow users to tap on a food item to view more detailed information. A subtle shadow effect can be added to each card to give the impression of depth.
- Detailed View (when a card is tapped): Tapping on a card should open a detailed view of the food item. This screen should include:
- Larger Image: A larger image of the food item.
- Full Nutritional Information: A detailed breakdown of all nutritional information, presented with the visual elements (progress bars, pie charts, gauges, sugar cubes) mentioned earlier.
- Ingredients List: A list of ingredients.
- Allergen Information: Information about potential allergens.
- Restaurant Information: A link to the restaurant’s location on a map or the option to order online.
- User Interface Elements: The illustration should include common UI elements like buttons, checkboxes, and sliders. These elements should be designed consistently with the overall aesthetic of the app.
- Color Scheme: The color scheme should be clean and inviting. Using a combination of bright and contrasting colors, such as the restaurant’s brand colors, and neutral backgrounds.
- Font and Typography: The illustration should use a clear, readable font for all text elements. Font sizes should be appropriate for easy reading on a mobile device.
Future Enhancements and Scalability
The “Fast Food Chooser” application, designed to simplify and enhance the fast-food selection process, is not a static entity. It’s a dynamic platform that must evolve to meet user needs, adapt to market changes, and maintain its relevance. This necessitates a forward-thinking approach to future enhancements and scalability. A robust plan will ensure the application’s longevity and continued success.
Potential Future Features and Enhancements
Expanding the “Fast Food Chooser” involves incorporating several enhancements that would improve user experience and functionality. These improvements can be categorized into several key areas: personalization, social integration, and enhanced data analysis.
- Advanced Personalization: Enhancements will enable more refined user profiles. This includes integrating dietary restrictions (e.g., vegan, gluten-free, low-carb), allergy information, and preferred cuisines. Users could specify their preferred price range, portion sizes, and even calorie targets. Furthermore, the application could learn user preferences over time using machine learning algorithms, tailoring recommendations based on past choices and feedback. For instance, if a user consistently selects healthier options, the algorithm would prioritize restaurants and menu items aligned with that preference.
- Social Integration: Allow users to share their fast-food choices and reviews with friends. Integrate social features like rating restaurants, commenting on menu items, and creating and sharing “favorite lists.” Implement a feature that allows users to see what their friends are eating or recommend. This will facilitate a social community aspect, fostering user engagement and driving application usage.
- Real-Time Order Tracking: Integration with third-party delivery services to provide real-time order tracking. This would give users up-to-the-minute updates on the status of their orders, from preparation to delivery. This feature is already common in many food delivery applications and provides users with a convenient experience.
- Voice Control: Implementing voice control for hands-free operation, using voice assistants such as Google Assistant or Siri. This would allow users to search for restaurants, filter menu items, and place orders using voice commands.
- Augmented Reality (AR) Features: Developing AR features that allow users to visualize menu items in their real-world environment. This could involve overlaying images of food items onto a table using a smartphone’s camera.
Expansion to Other Food Choices
The “Fast Food Chooser” concept can be expanded beyond fast food to encompass a broader range of food choices. This expansion would significantly increase the application’s utility and user base.
- Grocery Store Integration: Expand the application to include grocery stores. This would involve integrating data from grocery stores, including product information, pricing, and availability. Users could search for ingredients for recipes, compare prices between different stores, and create shopping lists.
- Restaurant Recommendations Beyond Fast Food: Include full-service restaurants, casual dining, and local eateries. This would involve integrating data from restaurant review websites and databases, such as Yelp and TripAdvisor. Users could search for restaurants based on cuisine, price range, and ratings.
- Recipe Integration: Integrate recipe databases, allowing users to find recipes based on their dietary preferences and available ingredients. The application could then suggest restaurants that offer similar dishes or suggest grocery store items for preparation.
- Meal Planning Features: Provide tools for meal planning, allowing users to create weekly meal plans based on their preferences and dietary needs. This feature could integrate with grocery store integrations to automatically generate shopping lists.
Scalability Requirements for the Application
As the user base grows, the “Fast Food Chooser” must scale to handle increased traffic, data volume, and processing demands. This requires careful consideration of the application’s architecture and infrastructure. Scalability considerations are critical for ensuring a smooth user experience.
- Database Scalability: The application’s database must be designed to handle a large volume of data. This includes restaurant information, menu items, user profiles, and order history. Implement database sharding, which involves distributing data across multiple servers to improve performance and reduce the load on individual servers.
- Server Infrastructure: The server infrastructure must be able to handle increased traffic. Utilize cloud-based hosting services, such as Amazon Web Services (AWS) or Google Cloud Platform (GCP), to scale the server resources dynamically. This allows the application to automatically adjust to traffic spikes.
- Caching Mechanisms: Implement caching mechanisms to reduce the load on the database and servers. Caching involves storing frequently accessed data in memory to speed up retrieval.
- Load Balancing: Implement load balancing to distribute traffic across multiple servers. Load balancing ensures that no single server is overloaded, improving application performance and availability.
- Code Optimization: Optimize the application’s code to improve performance. This includes optimizing database queries, minimizing the use of complex algorithms, and using efficient data structures.
Closure
So, there you have it – a glimpse into the world of the fast food chooser. From initial concept to potential future expansions, this tool is all about empowering you to make better food choices, whether you’re craving a quick bite or looking for something a little healthier. It’s not just about what you eat; it’s about how you eat. This tool has the potential to transform how we approach fast food, making it a more informed, personalized, and enjoyable experience for everyone.
Time to ditch the food guilt and embrace the possibilities!